Sibyl: Explaining Machine Learning Models for High-Stakes Decision Making
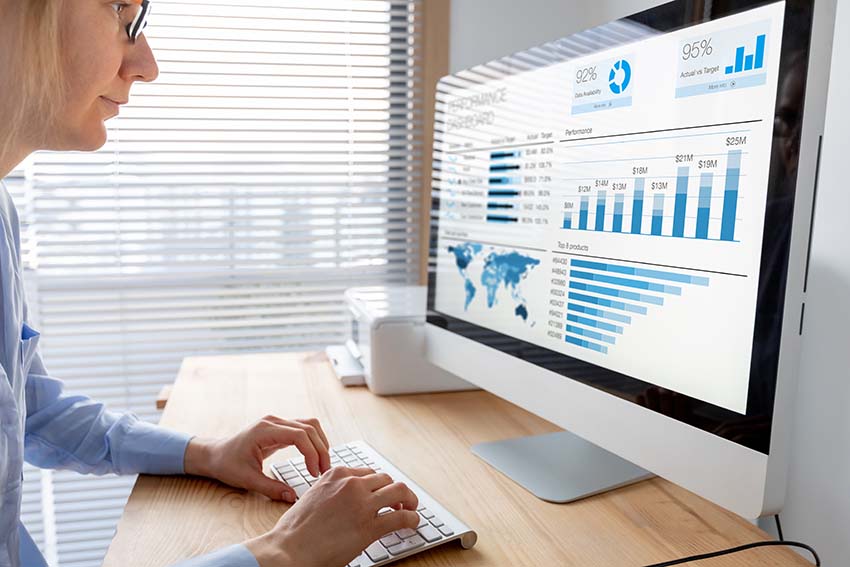
May-2021
This paper, presented at the CHI Conference on Human Factors in Computing Systems (May 2021), addresses the need for an effective way to explain machine learning predictions in the domain of child welfare screening. In child welfare, machine learning can be used to generate useful insights from the large amount of data available to screeners, potentially improving the outcomes for children referred for alleged abuse and neglect. Through a series of interviews and user studies, the authors developed Sibyl, a machine learning explanation dashboard. They tested four different explanation types and decided a local feature contribution approach was most useful to screeners.
Citation:
Zytek, A., Liu, D., Vaithianathan, R., & Veeramachaneni, K. (2021, May). Sibyl: Explaining Machine Learning Models for High-Stakes Decision Making. In Extended Abstracts of the 2021 CHI Conference on Human Factors in Computing Systems (pp. 1-6).
Using a Machine Learning Tool to Support High-Stakes Decisions in Child Protection
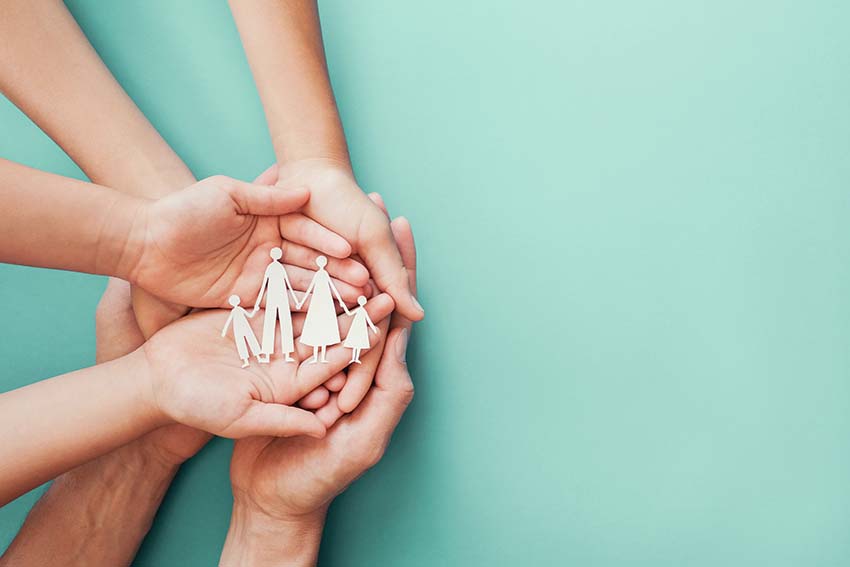
April-2021
While machine learning tools are becoming more widely used to support decisions in social domains, including child welfare, these tools need to be designed in a way that considers the interactions between the tools and humans. In this AI Magazine article the authors argue that human-centred design is key to the successful deployment and impact of machine learning tools. The Allegheny Family Screening Tool (AFST), a decision support tools used in Allegheny County (PA, United States) since 2016 to support child welfare call screening, is used as an example of human-centred design. The authors explain aspects of human-centred design that contributed to its successful deployment, including agency leadership and ownership, transparency by design, ethical oversight, community engagement, and social license. The authors conclude with potential next-steps for human-centred design in the development and implementation of machine learning decision support tools.
Citation:
Vaithianathan, R., Benavides-Prado, D., Dalton, E., Chouldechova, A., & Putnam-Hornstein, E. (2021). Using a Machine Learning Tool to Support High-Stakes Decisions in Child Protection. AI Magazine, 42(1), 53-60.
Adversities of Childhood Experience and School Readiness - Focus on children born to teen and non-teen mothers in the Growing Up in New Zealand data
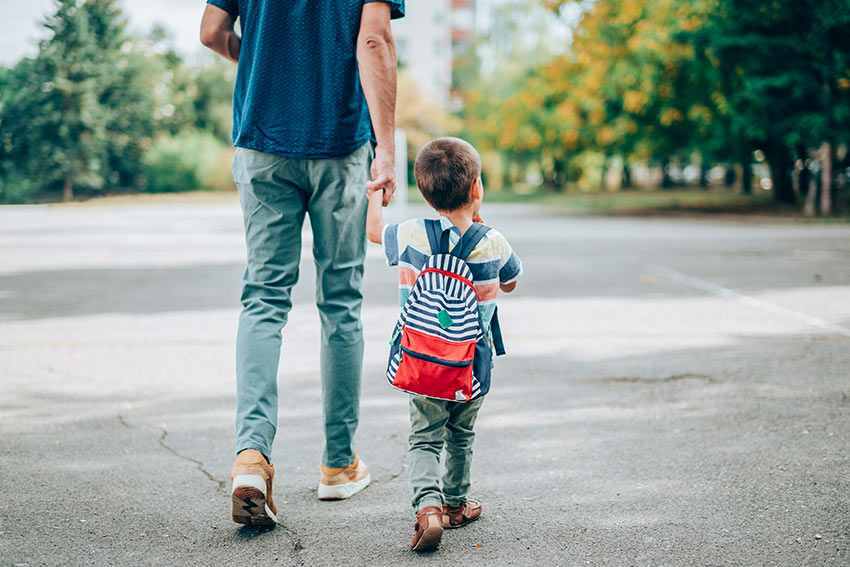
April-2021
This research looked at the prevalence of Adverse Childhood Experiences (ACEs) among children of teen mothers within the Growing Up in New Zealand (GUiNZ) cohort, and the extent to which the number of ACEs experienced relates to a child’s school readiness.
The researchers found ACES are more commonly experienced by children of teen mothers and observed a negative relationship between an increasing number of ACEs and a child’s school readiness. However, as researchers found poorer performance on school readiness tests at all levels of ACEs exposure, this suggests that children born to teen mothers might benefit from support to improve school readiness, even if observed ACE scores are low.
Citation:
Walsh, M.C., Maloney, T., Vaithianathan, R., & Pereda-Perez, P. (2021). Adversities of Childhood Experience and School Readiness - Focus on children born to teen and non-teen mothers in the Growing Up in New Zealand data. [PDF] Wellington: Ministry of Social Development.
School Readiness, Adversities in Childhood Experience and Access to Government Services: A Scoping Study on Potential Protective Factors
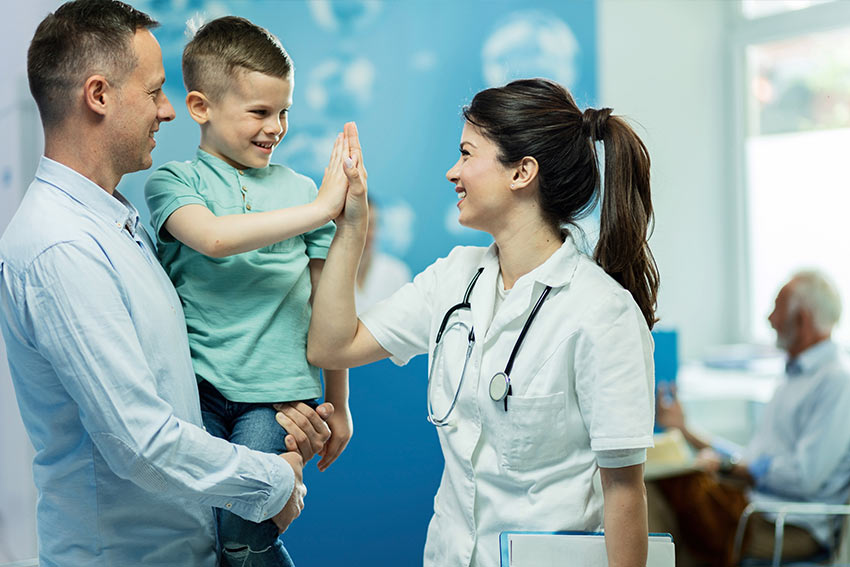
April-2021
This scoping study explored the extent to which government services across three domains: Healthcare, Early Childhood Education and Social Services, might offset negative impacts of Adverse Childhood Experiences (ACEs) and improve school readiness. It used data from the Growing Up in New Zealand (GUiNZ) longitudinal study.
The researchers found that, for children who had experienced multiples ACEs, four factors had the largest potential effect on school readiness. All those factors related to a child’s access to a general practitioner. The researchers also note their findings suggest access to good quality, paid early childhood education may have a role to play in closing the gaps in school readiness for children who experience ACEs.
Citation:
Walsh, M.C., Maloney, T., Vaithianathan, R., & Pereda-Perez, P. (2021). School Readiness, Adversities in Childhood Experience and Access to Government Services: A Scoping Study on Potential Protective Factors. [PDF] Wellington: Ministry of Social Development.