Our publications
This page lists our latest reports and academic papers.
Sibyl: Explaining Machine Learning Models for High-Stakes Decision Making
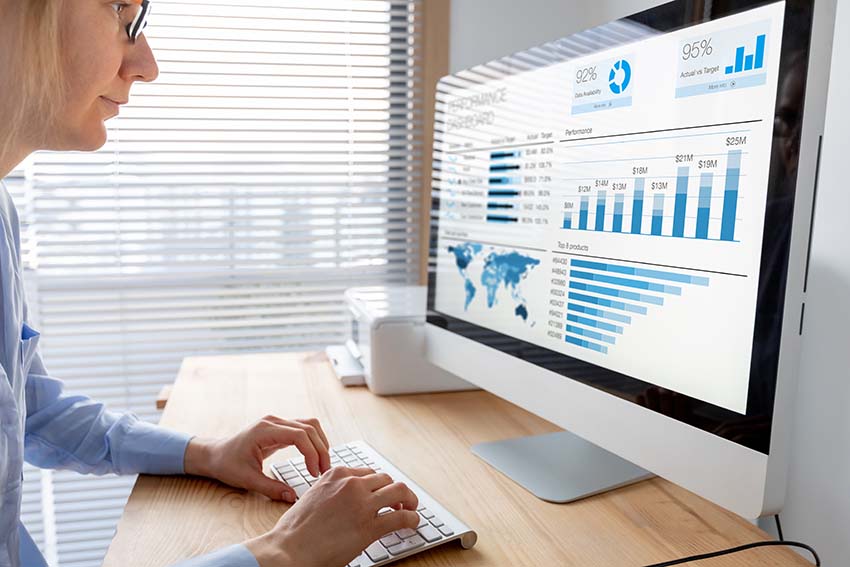
May-2021
This paper, presented at the CHI Conference on Human Factors in Computing Systems (May 2021), addresses the need for an effective way to explain machine learning predictions in the domain of child welfare screening. In child welfare, machine learning can be used to generate useful insights from the large amount of data available to screeners, potentially improving the outcomes for children referred for alleged abuse and neglect. Through a series of interviews and user studies, the authors developed Sibyl, a machine learning explanation dashboard. They tested four different explanation types and decided a local feature contribution approach was most useful to screeners.
Citation:
Zytek, A., Liu, D., Vaithianathan, R., & Veeramachaneni, K. (2021, May). Sibyl: Explaining Machine Learning Models for High-Stakes Decision Making. In Extended Abstracts of the 2021 CHI Conference on Human Factors in Computing Systems (pp. 1-6).
Using a Machine Learning Tool to Support High-Stakes Decisions in Child Protection
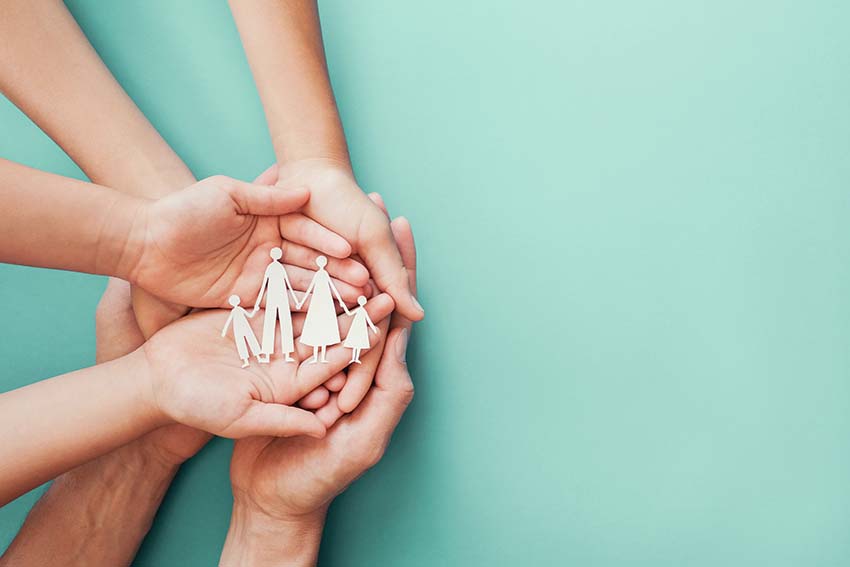
April-2021
While machine learning tools are becoming more widely used to support decisions in social domains, including child welfare, these tools need to be designed in a way that considers the interactions between the tools and humans. In this AI Magazine article the authors argue that human-centred design is key to the successful deployment and impact of machine learning tools. The Allegheny Family Screening Tool (AFST), a decision support tools used in Allegheny County (PA, United States) since 2016 to support child welfare call screening, is used as an example of human-centred design. The authors explain aspects of human-centred design that contributed to its successful deployment, including agency leadership and ownership, transparency by design, ethical oversight, community engagement, and social license. The authors conclude with potential next-steps for human-centred design in the development and implementation of machine learning decision support tools.
Citation:
Vaithianathan, R., Benavides-Prado, D., Dalton, E., Chouldechova, A., & Putnam-Hornstein, E. (2021). Using a Machine Learning Tool to Support High-Stakes Decisions in Child Protection. AI Magazine, 42(1), 53-60.
Adversities of Childhood Experience and School Readiness - Focus on children born to teen and non-teen mothers in the Growing Up in New Zealand data
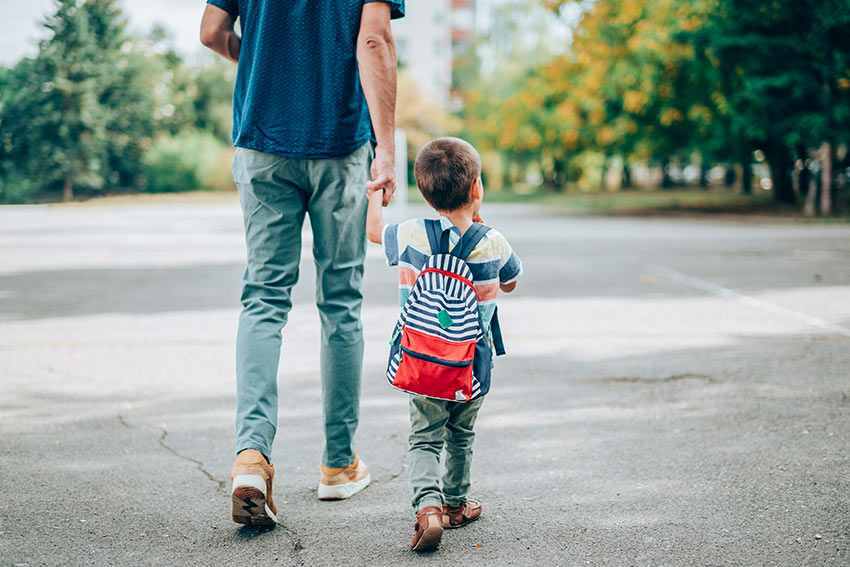
April-2021
This research looked at the prevalence of Adverse Childhood Experiences (ACEs) among children of teen mothers within the Growing Up in New Zealand (GUiNZ) cohort, and the extent to which the number of ACEs experienced relates to a child’s school readiness.
The researchers found ACES are more commonly experienced by children of teen mothers and observed a negative relationship between an increasing number of ACEs and a child’s school readiness. However, as researchers found poorer performance on school readiness tests at all levels of ACEs exposure, this suggests that children born to teen mothers might benefit from support to improve school readiness, even if observed ACE scores are low.
Citation:
Walsh, M.C., Maloney, T., Vaithianathan, R., & Pereda-Perez, P. (2021). Adversities of Childhood Experience and School Readiness - Focus on children born to teen and non-teen mothers in the Growing Up in New Zealand data. [PDF] Wellington: Ministry of Social Development.
School Readiness, Adversities in Childhood Experience and Access to Government Services: A Scoping Study on Potential Protective Factors
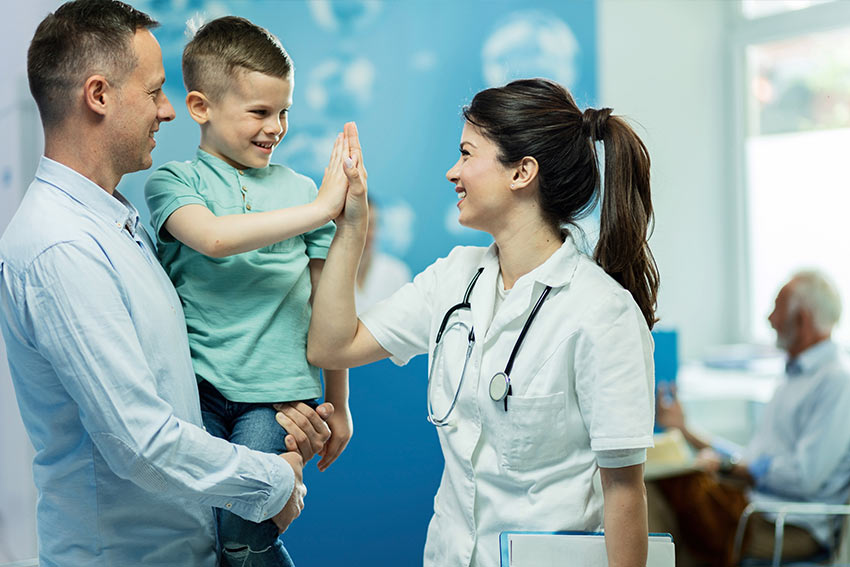
April-2021
This scoping study explored the extent to which government services across three domains: Healthcare, Early Childhood Education and Social Services, might offset negative impacts of Adverse Childhood Experiences (ACEs) and improve school readiness. It used data from the Growing Up in New Zealand (GUiNZ) longitudinal study.
The researchers found that, for children who had experienced multiples ACEs, four factors had the largest potential effect on school readiness. All those factors related to a child’s access to a general practitioner. The researchers also note their findings suggest access to good quality, paid early childhood education may have a role to play in closing the gaps in school readiness for children who experience ACEs.
Citation:
Walsh, M.C., Maloney, T., Vaithianathan, R., & Pereda-Perez, P. (2021). School Readiness, Adversities in Childhood Experience and Access to Government Services: A Scoping Study on Potential Protective Factors. [PDF] Wellington: Ministry of Social Development.
Measuring the working experience of doctors in training
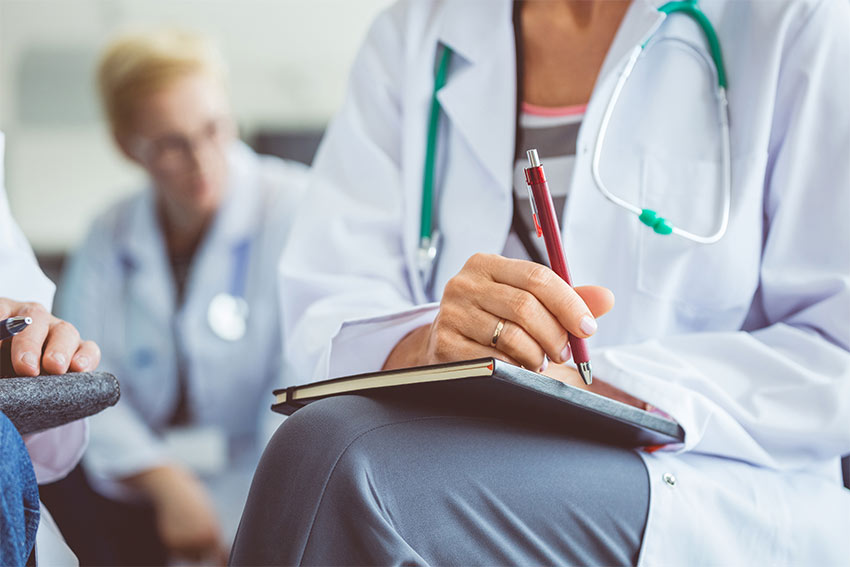
October-2020
In this paper published in the Future Healthcare Journal, researchers including CSDA’s Rhema Vaithianathan and Larissa Lorimer use an online tool (‘MyDay’) to look for an association between tasks and emotional affect for 565 doctors in training. They find that the participant trainee doctors spent a quarter of their time at work on paperwork or clinical work that did not involve patients, which was associated with more negative emotions. Positive emotions were associated with breaks, staff meetings, research, learning and clinical tasks that involved patients. Trainee doctors reporting that they had considered leaving medicine reported more negative feelings. The authors conclude that systematic workplace changes, like regular breaks, reduced paperwork and improved IT systems could contribute to positive workplace experiences and reduce the intention to quit among doctors in training.
Citation:
Hockey, P., Vaithianathan, R., Baeker, A., Beer, F., Goodall, A. H., Hammerton, M., Jarvis, R., Brock, S., & Lorimer, L. (2020). Measuring the working experience of doctors in training. Future Healthcare Journal, 7(3), e17–e22. https://doi.org/10.7861/fhj.2020-0005
Related projects:
MyDay Survey with Health Education England, Working Across Wessex
Implementing the Hello Baby Prevention Program in Allegheny County Methodology Report
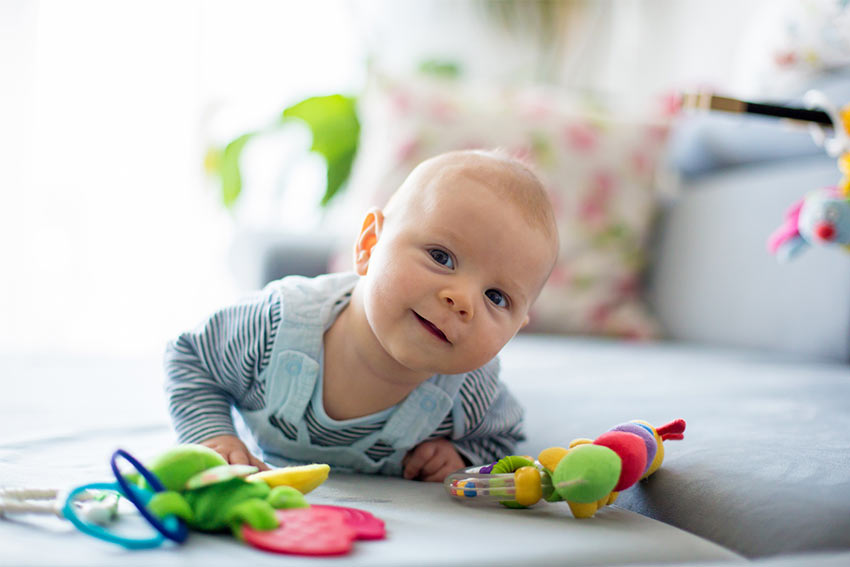
September-2020
This report summarises the development and implementation of the Hello Baby program which is supported by a predictive risk modelling (PRM) tool, built and deployed by the Centre for Social Data Analytics. Hello Baby is a new universal tiered program designed to prevent harm and protect children, introduced as a pilot program by the Allegheny County Department of Human Services in September 2020. The County will use the PRM tool to establish eligibility for higher intensity services, alongside self-referral and professional referral. The methodology provides background to the introduction of Hello Baby, explains the selection of the PRM tool as an eligibility pathway, describes how historical data was used to train and validate the PRM tool and explains how the tool will be used as part of the implemented Hello Baby program. Additional documentation about the Hello Baby program including ethics reports and FAQs is found on the Allegheny County website.
Citation:
Vaithianathan, Rhema; Diana Benavides-Prado and Emily Putnam-Hornstein. Implementing the Hello Baby Prevention Program in Allegheny County. Centre for Social Data Analytics. Auckland, New Zealand. September 2020.
Related projects:
Hello Baby Program - Proactive Child Welfare Predictive Risk Model
Using Predictive Risk Modeling to Prioritize Services for People Experiencing Homelessness in Allegheny County: Methodology Paper for the Allegheny Housing Assessment
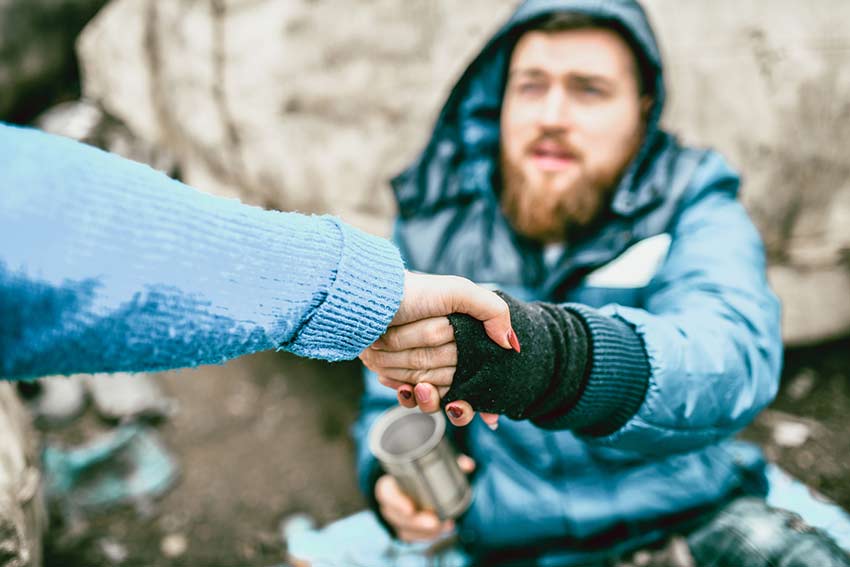
September-2020
This report summarises the development and implementation of the Allegheny Housing Assessment, a predictive risk modelling tool, built and deployed by the Centre for Social Data Analytics for the Allegheny County Department of Human Services, Pennsylvania, United States. The AHA will be used to support the prioritisation of people for longer term housing programs. A prioritisation tool is needed because the demand for housing exceeds supply. The AHA replaces the VI-SPDAT, a prioritisation system without local validation. The methodology provides background to the development of the AHA including a comparison between the AHA and the previously used VI-SPDAT. The methodology provides background on homeless services in Allegheny County and the decision to explore the use of a predictive risk modelling tool, before discussing elements including community engagement, the methodology used to develop the tool, accuracy and the business process it will be used within.
Citation:
Vaithianathan, R. & Kithulgoda, C.I. (2020). Using Predictive Risk Modeling to Prioritize Services for People Experiencing Homelessness in Allegheny County. Centre for Social Data Analytics. Auckland, New Zealand.
Related projects:
Hospital Injury Encounters of Children Identified by a Predictive Risk Model for Screening Child Maltreatment Referrals: Evidence from the Allegheny Family Screening Tool
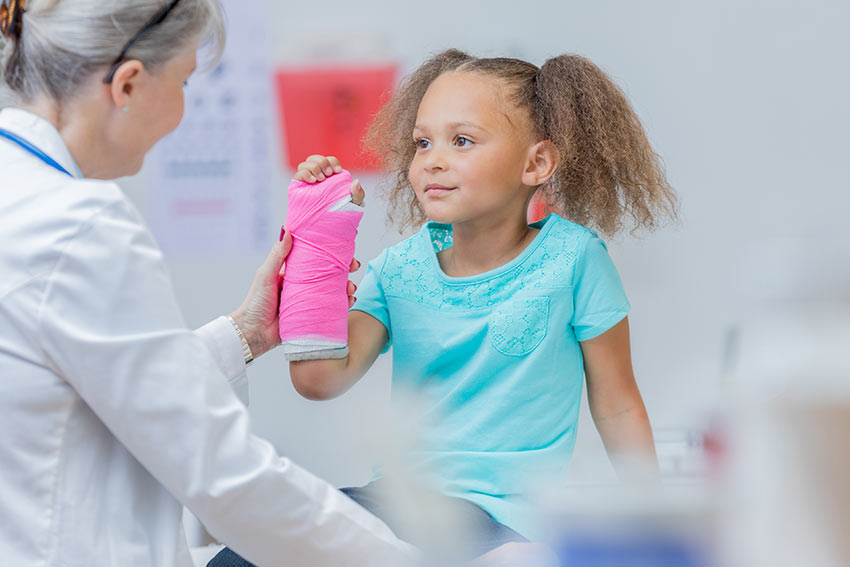
August-2020
This paper confirms that children identified as at risk by the Allegheny Family Screening Tool (AFST), a predictive risk modelling tool that supports child protection decisions in Allegheny County (PA, United States), are also at considerably heightened risk of hospitalisation injury. The authors, including Rhema Vaithianathan and Diana Benavides Prado, used a one-off linkage between the child protection system and Pittsburgh Children’s Hospital data to show that children identified as at risk by the AFST are indeed at considerably heightened risk of injury, abuse, and self-harm hospitalisation. The authors also identified a sharply heightened hospitalisation risk for children receiving the highest AFST scores and found that the AFST is particularly sensitive to the risk facing White children, which means the child protection system is potentially under protecting those children.
Citation:
Vaithianathan R, Putnam-Hornstein E, Chouldechova A, Benavides-Prado D, Berger R. Hospital Injury Encounters of Children Identified by a Predictive Risk Model for Screening Child Maltreatment Referrals: Evidence From the Allegheny Family Screening Tool. JAMA Pediatr. Published online August 03, 2020. doi:10.1001/jamapediatrics.2020.2770
Related projects:
Allegheny Family Screening Tool
Digital Contact Tracing for COVID-19: A Primer for Policymakers
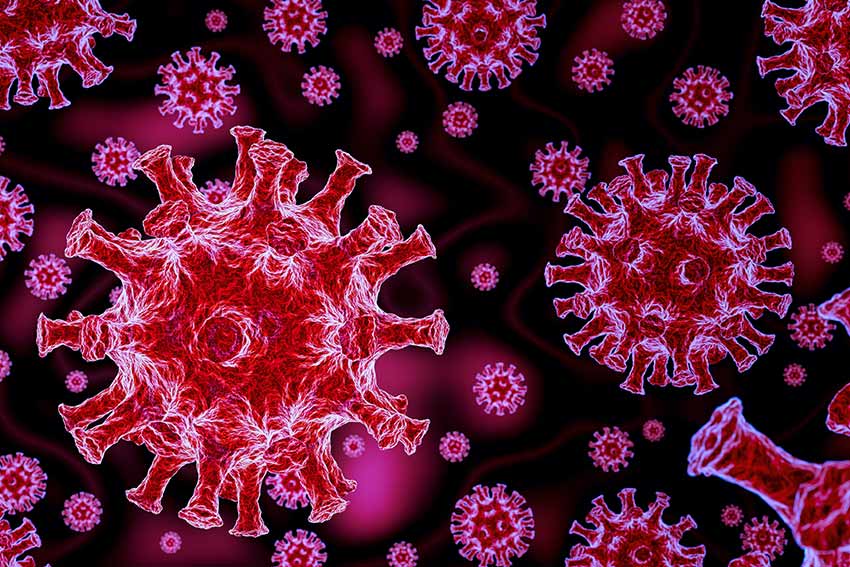
June-2020
This working paper is intended as a guide for policymakers considering the use of digital contact tracing solutions for Covid-19. The authors, including Rhema Vaithianathan and Nina Anchugina, use a simple graphical model of infection transmissions to illustrate why COVID-19 is particularly challenging to manage with traditional manual contact tracing. They find that digital contact tracing solutions for COVID-19 must offer exceptional speed and achieve high take-up rates to be useful. The paper discusses the importance of social licence for take-up, and explains how a commitment to impact evaluation can help build trust.
Citation:
Vaithianathan, R., Ryan, M., Anchugina, N., Selvey, L., Dare, T., & Brown, A. (2020). Digital Contact Tracing for Covid-19: A Primer for Policymakers (Working Paper). Centre for Social Data Analytics: Auckland University of Technology & The University of Queensland.
Related links
- 'Covid-19 Contact Tracing Apps: What works?'
presentation by Nina Anchugina for the Chief Data and Analytics Officer (CDAO) NZ conference, November 2020 (video) - COVIDSafe Still Has Crucial iPhone Issues Nearly 2 Months Later
Gizmodo, 16 June 2020. - Even 100% COVIDSafe Uptake Won’t Make It Effective, Researcher Says
Gizmodo, 11 June 2020. - COVIDSafe extremely limited’: New research
Innovation Aus, 9 June 2020. - Five ways New Zealand can keep Covid-19 cases at zero
The Guardian, 8 June 2020. - Trust in COVID Tracer app needs to be built to see sufficient uptake - Anna Brown
Newshub, 7 June 2020 - Covid 19 coronavirus: The three extra steps NZ needs to take at alert level 1
NZ Herald, 2 June 2020. - Coronavirus: Government app lacking crucial features – academic
Radio NZ, 21 May 2020. - Covid 19 coronavirus: Govt tracing app only good for 'one unlikely scenario' – academic
NZ Herald, 20 May 2020. - Covid 19 coronavirus: Rhema Vaithianathan: NZ is the Wild West of contact tracing
NZ Herald, 14 May 2020.
Towards Knowledgeable Supervised Lifelong Learning Systems
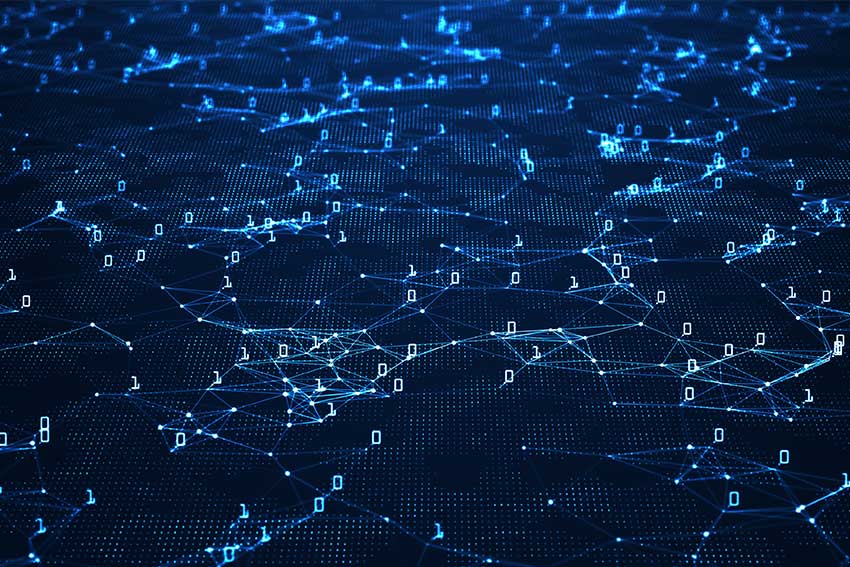
May-2020
This paper co-authored by Diana Benavides-Prado offers a potential solution to challenges associated with machine learning systems that learn sequentially. The authors propose a framework for long-term learning systems (Proficiente), which relies on transferring knowledge across tasks in two directions using Support Vector Machines (SVM). Proficiente makes it possible to transfer knowledge acquired from a previous task forward to a new target task and to transfer knowledge acquired from recent tasks backward to refine knowledge acquired from previous tasks. The authors demonstrate that transferring selected knowledge forward and backwards has the potential to encourage learning systems to become more knowledgeable while observing tasks sequentially.
Citation:
Benavides-Prado, D., Koh, Y. S., & Riddle, P. (2020). Towards Knowledgeable Supervised Lifelong Learning Systems. Journal of Artificial Intelligence Research, 68, 159-224.
Publications from previous years
Implementing a Child Welfare Decision Aide in Douglas County: Methodology Report
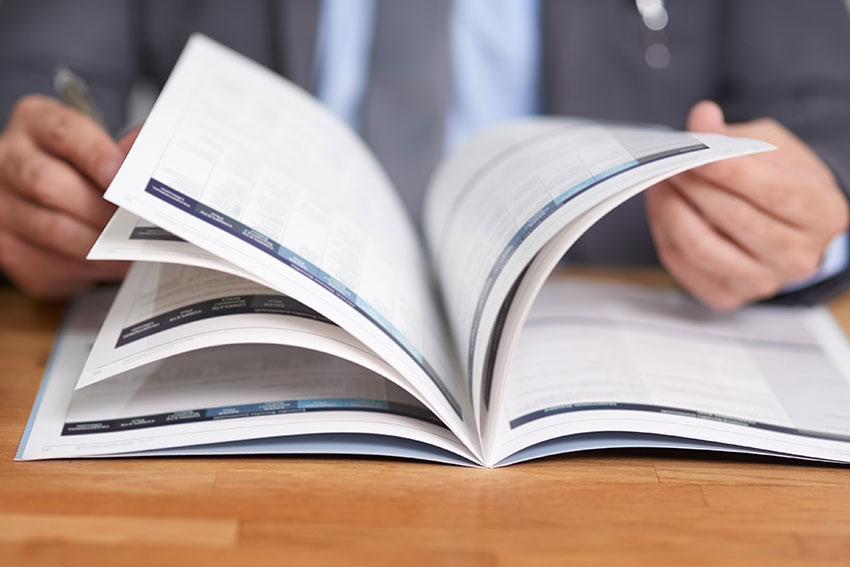
December-2019
This report summarises the development and implementation of the Douglas County Decision Aide (DCDA), a child welfare predictive risk-modelling tool built and deployed by the Centre for Social Data Analytics. The DCDA is intended to support call screening of child maltreatment referrals made to the Douglas County Department of Human Services (CO, United States). The methodology outlines how Douglas County was triaging referrals prior to the deployment of the DCDA and how the DCDA has changed that, as well as details of how the DCDA tool was built and validated and how ethical concerns were identified and responded to.
Citation:
Vaithianathan, R., Dinh, H., Kalisher, A., Kithulgoda, C. I., Kulick, E., Mayur, M., … Putnam-Hornstein, E. (December 2019). Implementing a Child Welfare Decision Aide in Douglas County: Methodology Report. Centre for Social Data Analytics.
Related Projects:
Douglas County Decision Aide
Exploring the protective factors of children and families identified at highest risk of adverse childhood experiences by a predictive risk model: An analysis of the Growing Up in New Zealand cohort
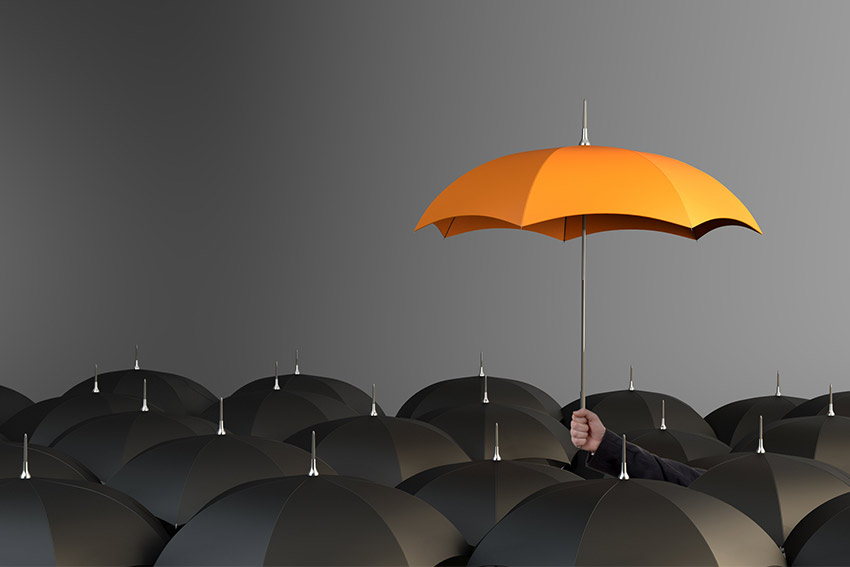
December-2019
The authors explore what protective factors might exist for the families of children identified by a predictive risk model as at high risk of experiencing adverse childhood experiences. Identifying protective factors is an important step in designing preventive services for families as well as helping social workers take a strengths-based approach to these families. The authors identify 56 factors associated with protective effects against adversities and find that a positive mother-partner relationship helps children at risk of adversities.
Citation:
Walsh, M. C., Joyce, S., Maloney, T., & Vaithianathan, R. (2019). Exploring the Protective Factors of Children and Families Identified at Highest Risk of Adverse Childhood Experiences by a Predictive Risk Model: An Analysis of the Growing up in New Zealand Cohort. Children and Youth Services Review, 104556.
Related Projects:
Adverse Childhood Experiences, Protective Factors and School Readiness
Indigenous voices on measuring and valuing health states
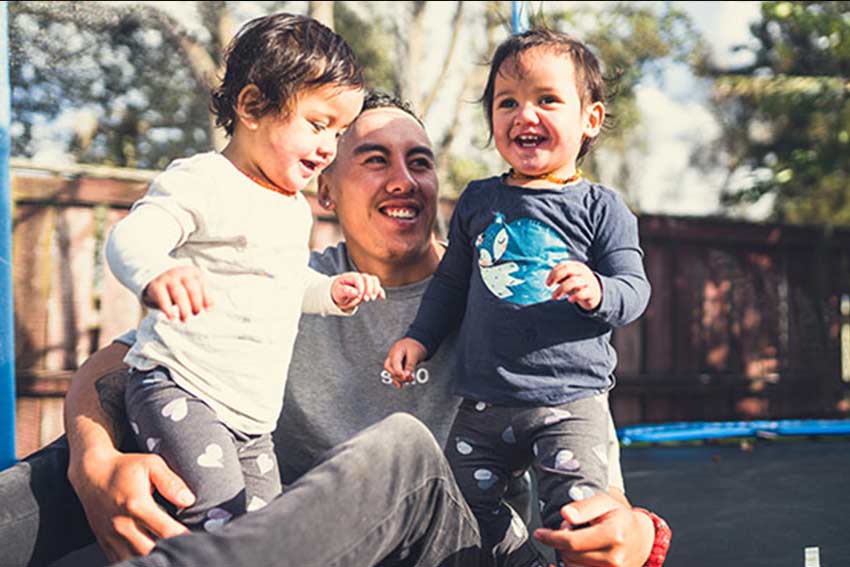
November-2019
This article sets out to start a conversation around what an Indigenous measure of health might look like and how it might value key dimensions of health. Within a Kaupapa Māori theoretical paradigm, in-depth interviews were conducted with six Māori key informants who had cared for whānau (family) members through illness to give voice to dimensions of health and illness that Western economic measures of health fail to capture.
Citation:
Willing, E., Paine, S. J., Wyeth, E., Te Ao, B., Vaithianathan, R., & Reid, P. (2019). Indigenous voices on measuring and valuing health states. AlterNative: An International Journal of Indigenous Peoples, 1177180119885418.
Ethnic Disparities in Childhood Prevalence of Maltreatment: Evidence from a New Zealand Birth Cohort
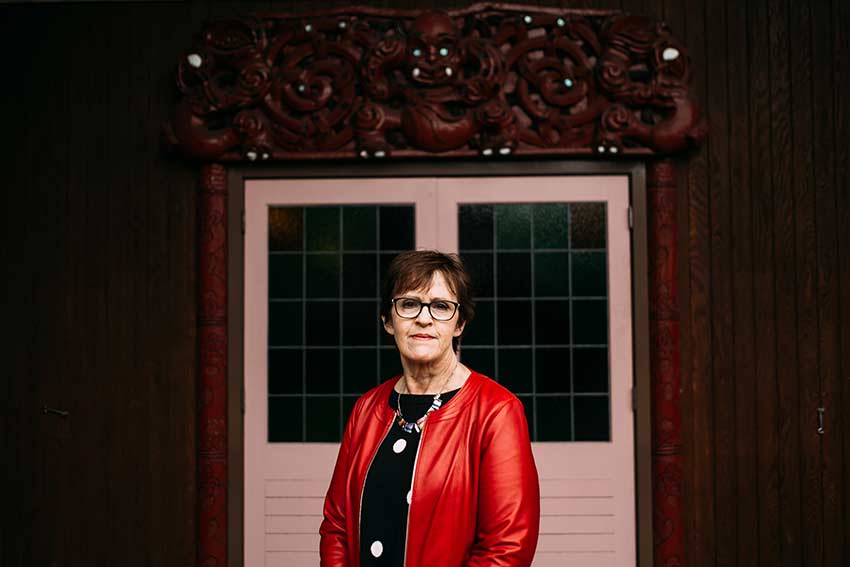
July-2019
This article co-authored by Rhema Vaithianathan and Benedicte Rouland (CSDA research fellow 2016-2018) aims to document ethnic disparities in childhood abuse and neglect among New Zealand children. By following the 1998 New Zealand birth cohort of 56,904 children through 2016, the authors identify substantial ethnic differences in child maltreatment and child protection involvement. They find that despite long-standing child support policies and reparation for breaches of Indigenous people’s rights, significant child maltreatment disparities persist.
Citation:
Rouland, B., Vaithianathan, R., Wilson, D., & Putnam-Hornstein, E. (2019). Ethnic Disparities in Childhood Prevalence of Maltreatment: Evidence From a New Zealand Birth Cohort. Am J Public Health.
Related Project:
Ethnic Disparities in Child Protection
Adverse childhood experiences and school readiness outcomes: Results from the GUiNZ study
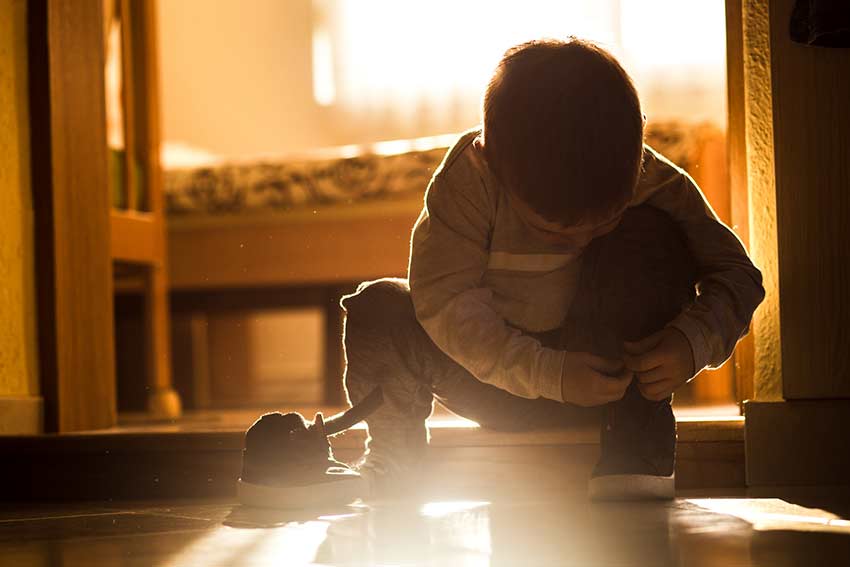
April-2019
This report describes using Growing Up in New Zealand (GUiNZ) survey instruments to create a measurement of adverse child experiences (ACEs) and correlates this measurement with school readiness outcomes. Statistically significant associations were found between a child’s experience of ACEs and their performance in cognitive tests administered at 54 months. This study was authored by Matthew Walsh, Sophie Joyce, Tim Maloney and Rhema Vaithianathan and funded by the Children and Families Research Fund (Ministry of Social Development, NZ).
Citation:
Walsh, M. C., Maloney, T., Vaithianathan, R., & Joyce, S. (2019). Adverse childhood experiences and school readiness outcomes: Results from the Growing up in New Zealand study. Wellington: Ministry of Social Development.
Related Projects:
Adverse Childhood Experiences, Protective Factors and School Readiness
Protective factors of children and families at highest risk of adverse childhood experiences: An analysis of children and families in the Growing up in New Zealand data who “beat the odds”
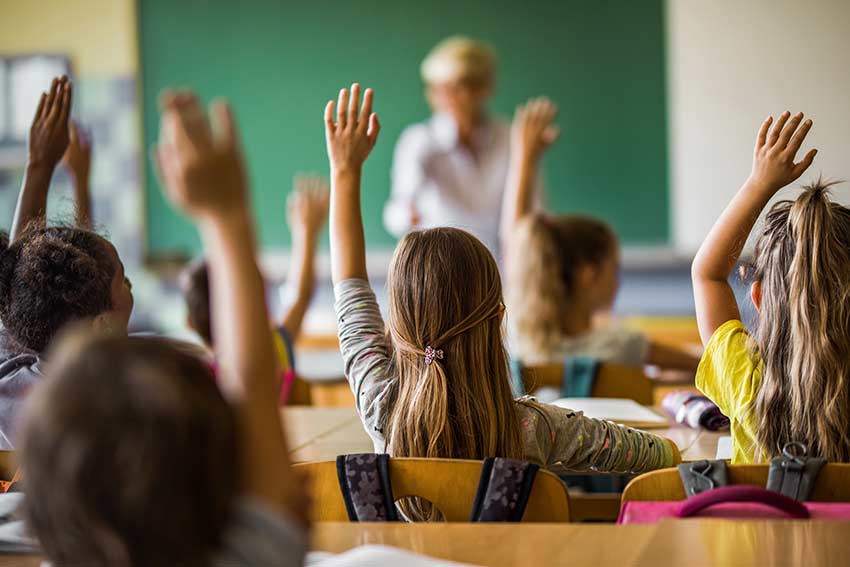
April-2019
With increasing access to integrated administrative data, it is easy to identify infants who are likely to suffer childhood adversities. However, many infants who appear “at risk” end up thriving - experiencing few of the adversities that beset other children with similar risk factors. Understanding what helps children “beat the odds” is important for policymakers and frontline services that want to help families at risk. This report authored by Matthew Walsh, Tim Maloney (CSDA co-director 2016-2019), Rhema Vaithianathan and Sophie Joyce analyses the Growing Up in New Zealand (GUiNZ) birth cohort to identify protective factors for at-risk children who “beat the odds”.
Citation:
Walsh, M. C., Maloney, T., Vaithianathan, R., & Joyce, S. (2019). Protective factors of children and families at highest risk of adverse childhood experiences: An analysis of children and families in the Growing up in New Zealand data who "beat the odds". Wellington: Ministry of Social Development.
Related Projects:
Adverse Childhood Experiences, Protective Factors and School Readiness
Adverse childhood experiences and school readiness outcomes: Results from the Growing up in New Zealand study
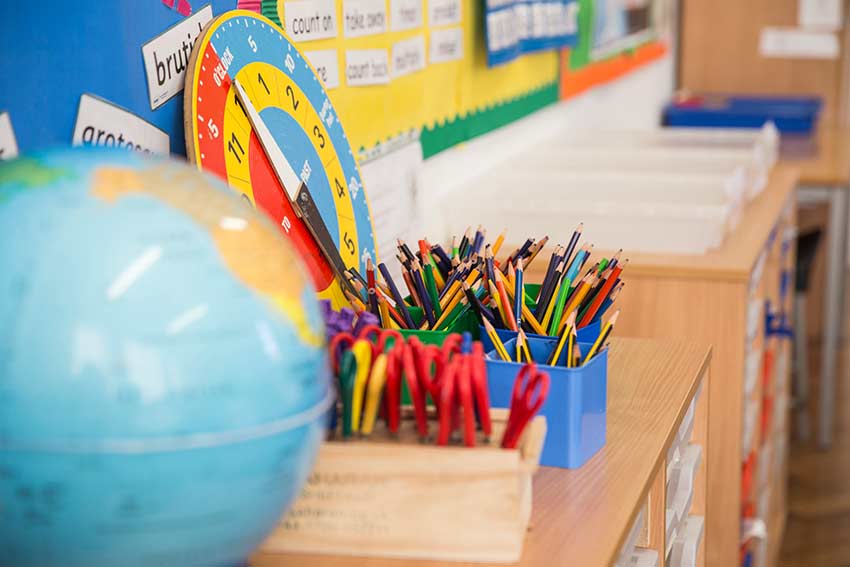
April-2019
The Center for Disease Control’s (CDC) Adverse Childhood Experiences (ACEs) have been associated with adverse health consequences in adults and children, but less is known about any association between ACEs and early learning skills. Researchers Matthew Walsh, Sophie Joyce, Tim Maloney and Rhema Vaithianathan investigated the relationship between ACEs and objective preschool measures of skills using the Growing up in New Zealand (GUiNZ) cohort study.
Citation:
Walsh, M. C., Joyce, S., Maloney, T., & Vaithianathan, R. (2019). Adverse childhood experiences and school readiness outcomes: results from the Growing up in New Zealand study. The New Zealand Medical Journal, 132(1493), 15-24.
Related Projects:
Adverse Childhood Experiences, Protective Factors and School Readiness
Toward Algorithmic Accountability in Public Services: A Qualitative Study of Affected Community Perspectives on Algorithmic Decision-making in Child Welfare Services
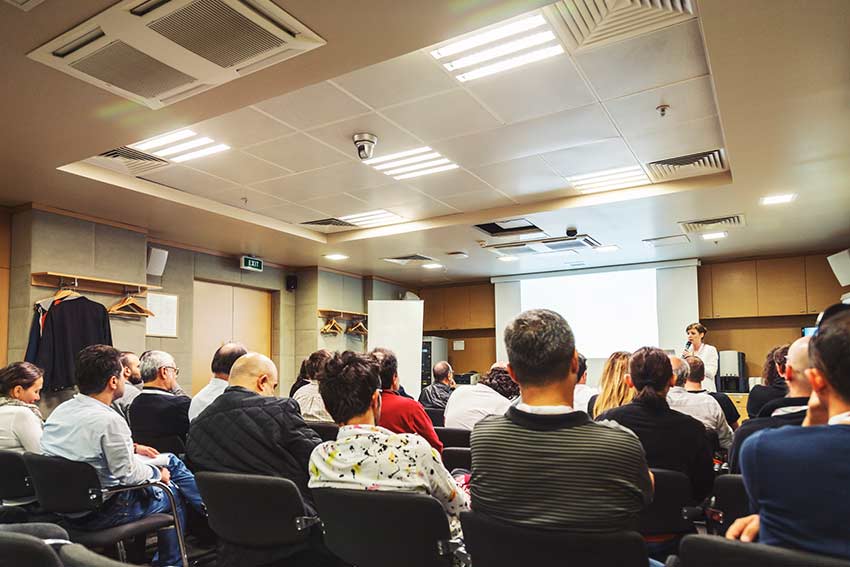
April-2019
Researchers, policy experts, and civil rights groups have all voiced concerns that algorithmic decision-making systems are being deployed without adequate consideration of potential harms, disparate impacts, and public accountability practices. Yet little is known about the concerns of those most likely to be affected by these systems. The authors, including Rhema Vaithianathan report and discuss the findings of workshops conducted to learn about the concerns of affected communities in the context of child welfare services.
Citation:
Brown, A., Chouldechova, A., Putnam-Hornstein, E., Tobin, A., & Vaithianathan, R. (2019, April). Toward Algorithmic Accountability in Public Services: A Qualitative Study of Affected Community Perspectives on Algorithmic Decision-making in Child Welfare Services. In Proceedings of the 2019 CHI Conference on Human Factors in Computing Systems (p. 41). ACM.
Related Project:
Community Perspectives on the Use of Algorithms by Government
Allegheny Family Screening Tool: Methodology, Version 2
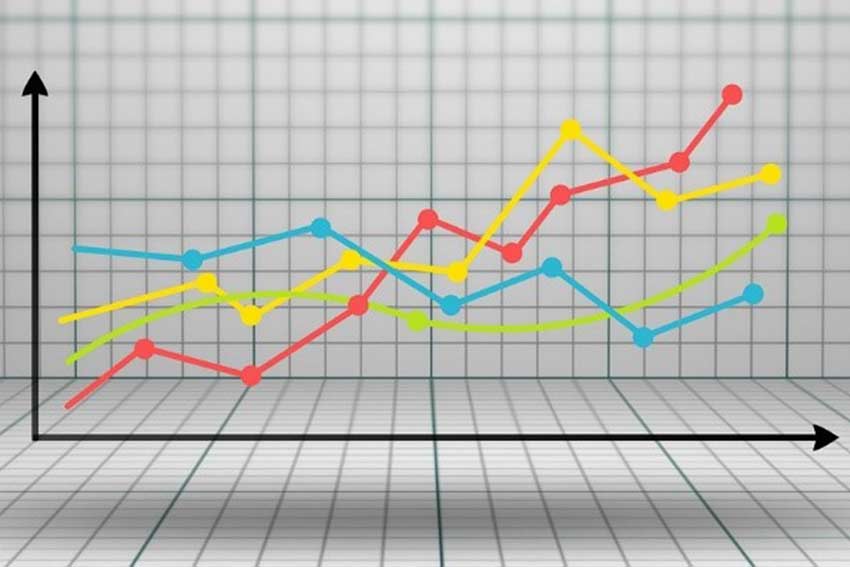
February-2019
This methodology report describes changes to the Allegheny Family Screening Tool (AFST), building upon and updating the original methodology report, Developing Predictive Risk Models to Support Child Maltreatment Hotline Screening Decisions (March 2017). Modifications implemented include changes to specific predictor fields used in the model itself, the modelling methodology, and County policies concerning the tool’s use, upholding Allegheny County’s ongoing commitment to transparency by continuing to inform the community about changes to the tool and the County’s policies.
Citation:
Vaithianathan, R., Kulick, E., Putnam-Hornstein, E., & Benavides-Prado, D. (2019). Allegheny Family Screening Tool: Methodology, Version 2. Auckland: Centre for Social Data Analytics.
Related Project:
Allegheny Family Screening Tool
He awa whiria—braided rivers: Understanding the outcomes from Family Start for Māori
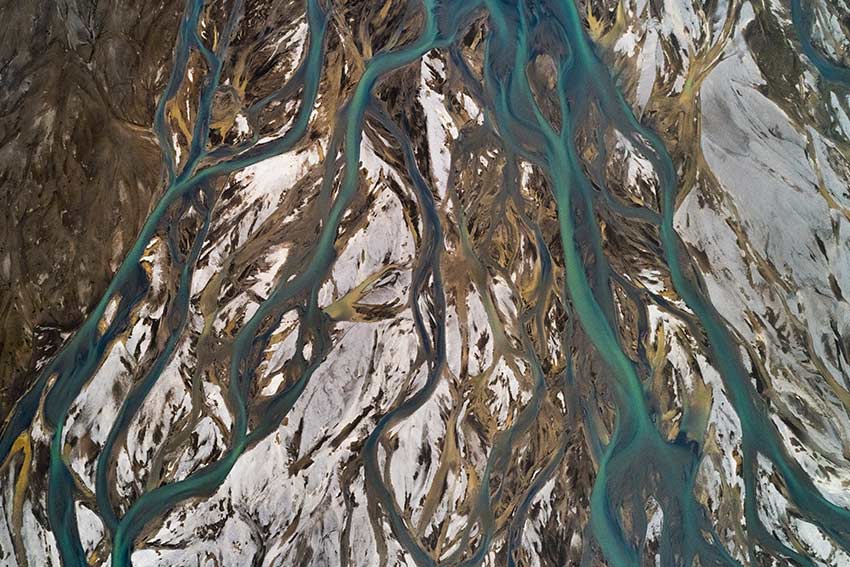
November-2018
In Aotearoa, New Zealand, the “braided rivers—he awa whiria” metaphor is facilitating conversations between Māori (indigenous peoples) and non-Māori researchers about the integration of knowledge systems. Rhema Vaithianathan and her co-authors explore how an approach based on he awa whiria can work in practice, in the examination of the efficacy for Māori whānau (families) of the government’s intensive home-visiting programme; Family Start. Published in Evaluation Matters—He Take Tō Te Aromatawai Online First.
Citation:
Cram, F., Vette, M., Wilson, M., Vaithianathan, R., Maloney, T., & Baird, S. (2018). He awa whiria—braided rivers: Understanding the outcomes from Family Start for Maori. Evaluation Matters, 165-207.
Related Project:
Family Start impact evaluation
Estimating the economic costs of ethnic health inequalities: protocol for a prevalence-based cost-of-illness study in New Zealand (2003-2014)
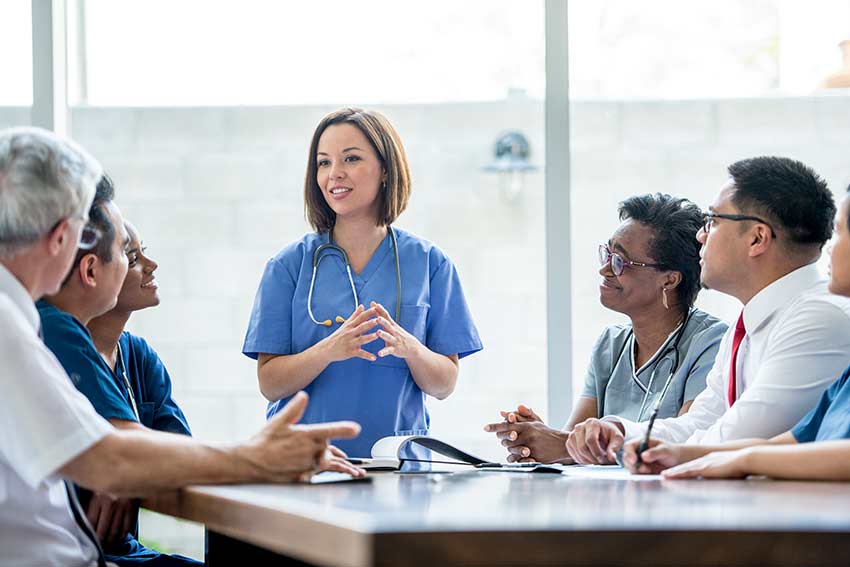
June-2018
This paper is a protocol for a proposed study, by researchers including Rhema Vaithianathan, that will investigate inequities in health between the indigenous Māori and non-Māori adult population in New Zealand and estimate the economic costs associated with these differences.
Citation:
Reid, P., Paine, S., Te Ao, B., Vaithianathan, R., Willing, E., & Wyeth, E. (2018, June). Estimating the Economic Costs of Ethnic Health Inequalities: Protocol for a Prevalence-Based-Cost-of-Illness-Study in New Zealand (2003-2014). BMJ Open, 8(6), e020763.
The impact of Social Workers in Schools: A preliminary investigation using linked administrative data
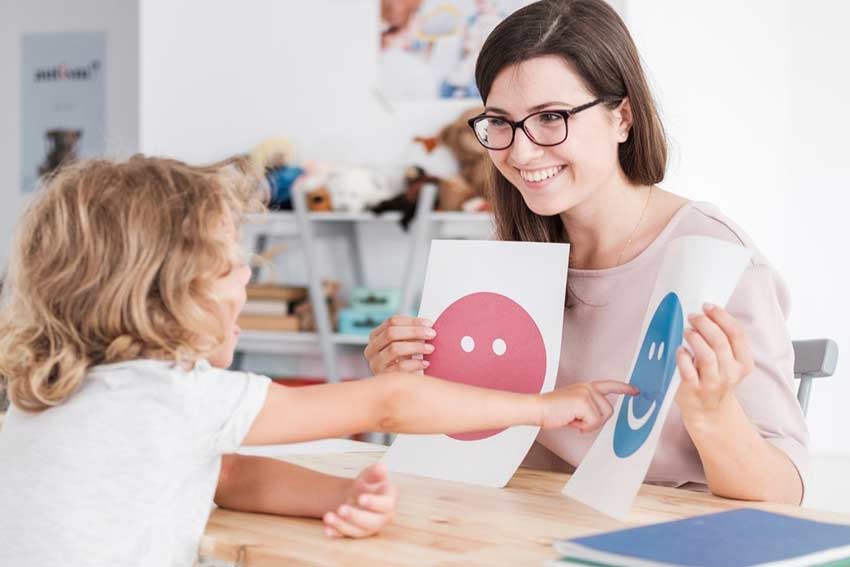
April-2018
This paper co-authored by Rhema Vaithianathan and Tim Maloney (CSDA co-director 2016-2019) reports on a preliminary investigation of the impact of the government-funded Social Workers in Schools service (SWiS) which is available in selected primary and intermediate schools. The aim of SWiS is to see safe, healthy and socialised children with a strong sense of identity, who are fully engaged in school. This analysis compares outcomes for children who attended SWiS schools with similar children who attended similar schools that at the time were not part of the SWiS programme. The study finds evidence of a reduction in non-enrolment days, a 5% increase for girls attaining NCEA level 1 by their 16th birthday, and a reduction in CYF youth justice referrals for the first three years of high school.
Citation:
Jiang, N., Maloney, T., Staneva, A., Wilson, M., & Vaithianathan, R. (2018). The impact of Social Workers in Schools: A preliminary investigation using linked administrative data.
Related Project:
Social Workers in Schools Impact Evaluation
Labour market effects of activating sick-listed workers
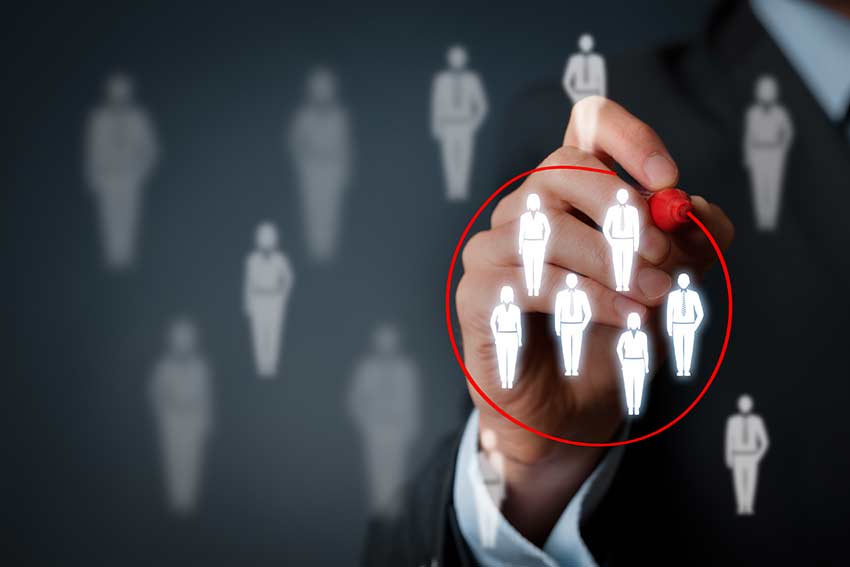
April-2018
Bénédicte Rouland (CSDA research fellow 2016-2018) and her co-authors use data from a large-scale randomized controlled trial conducted in Danish job centres to investigate the effects of activating sick-listed workers on subsequent labour market outcomes. Comparing treated and controls, the authors find an overall unfavourable effect on subsequent labour market outcomes.
Citation:
Rehwald, K., Rosholm, M., & Rouland, B. (2018). Labour market effects of activating sick-listed workers. Labour Economics, 53, 15-32.
Cumulative prevalence of maltreatment among New Zealand children, 1998–2015
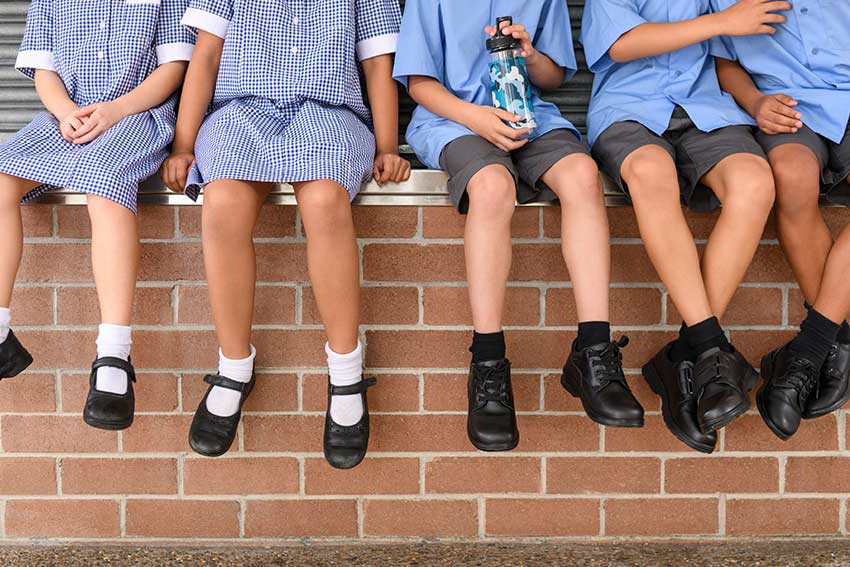
April-2018
Bénédicte Rouland (CSDA research fellow 2016-2018) and Rhema Vaithianathan explore the cumulative prevalence in New Zealand of notifications to child protective services, substantiated maltreatment cases and out-of-home placements. The study shows that one in four New Zealand children will be subject to at least one notification by age 17, an incidence of notification higher than that of medicated asthma among children.
Citation:
Rouland, B., & Vaithianathan, R. (2018). Cumulative prevalence of maltreatment among New Zealand children, 1998–2015.American Journal of Public Health, 108(4), 511-513.
A case study of algorithm-assisted decision making in child maltreatment hotline screening decisions
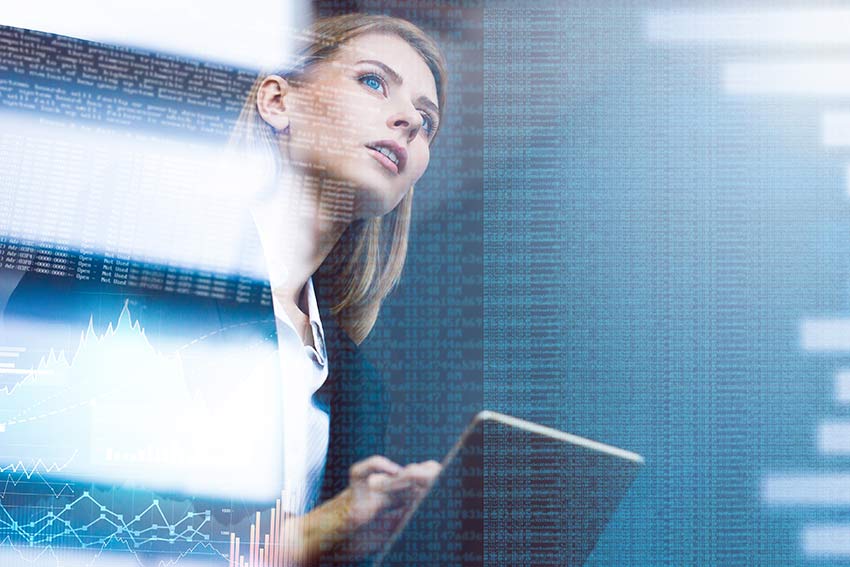
March-2018
Diana Bendavides-Prado, Rhema Vaithianathan, Oleksandr Fialko (CSDA research fellow 2017-2018), Alexandra Chouldechova and Emily Putnam-Hornstein describe their work on developing, validating, fairness auditing, and deploying a risk prediction model in Allegheny County, PA, USA. They discuss the results of their analysis to-date and highlight critical problems and data bias issues that present challenges for model evaluation and deployment.
Citation:
Chouldechova, A., Putnam-Hornstein, E., Benavides-Prado, D., Fialko, O., & Vaithianathan, R. (2018, January). A case study of algorithm-assisted decision making in child maltreatment hotline screening decisions. In Conference on Fairness, Accountability and Transparency (pp. 134-148).
Related Project(s):
Allegheny Family Screening Tool, Douglas County Decision Aide, California Child Welfare Predictive Risk Model Proof of Concept
Injury and mortality among children identified as at high risk of maltreatment
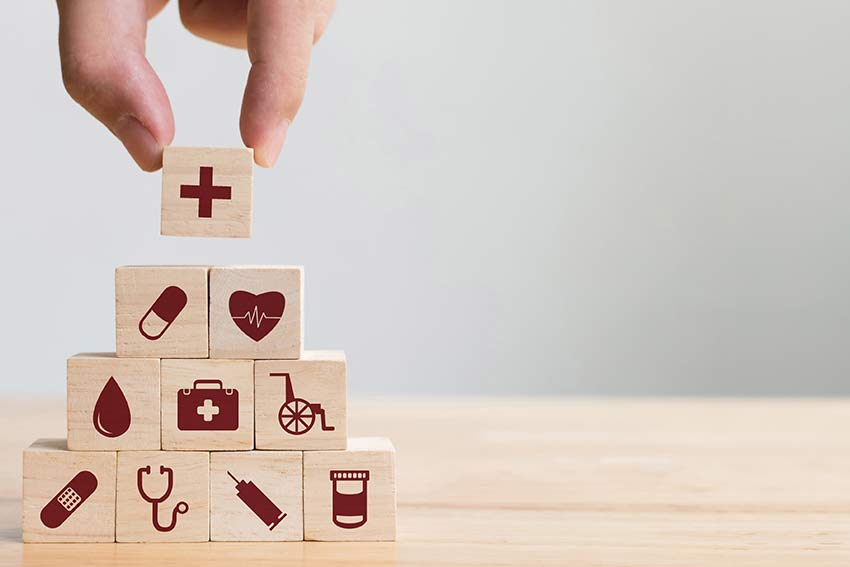
February-2018
This study by Rhema Vaithianathan, Bénédicte Rouland (CSDA research fellow 2016-2018) and Emily Putnam-Hornstein explored a model which assigned risk scores for the risk of a substantiated finding of maltreatment to children born in New Zealand in 2010, to see whether children at the highest 10% and 20% of risk would have an elevated chance of injury or death in early childhood. The study found that children assessed as being “very high risk” (highest 10%) and “high-risk” (highest 20%) had 4.8 times and 4.2 times respectively higher post-neonatal mortality rates than other children.
Citation:
Vaithianathan, R., Rouland, B., & Putnam-Hornstein, E. (2018). Injury and mortality among children identified as at high risk of maltreatment. Pediatrics, 141(2), e20172882.
Related Project(s):
Hello Baby Program - Proactive Child Welfare Predictive Risk Model
Risk assessment and decision making in child protective services: Predictive risk modeling in context
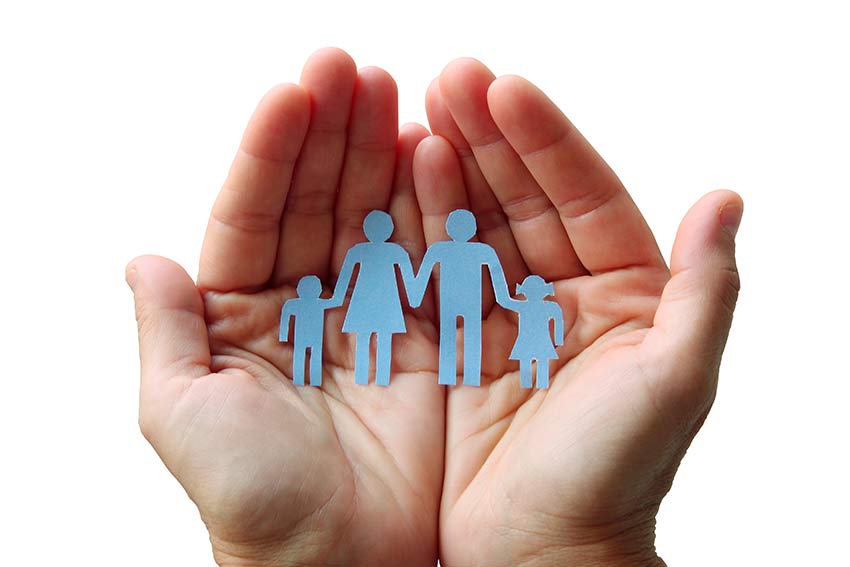
June-2017
The authors, including Rhema Vaithianathan, review the literature and provide a context for predictive risk modelling in the current risk assessment paradigm in child protective services. They describe how predictive analytics or predictive risk modelling using linked administrative data may provide a useful complement to current approaches.
Citation:
Cuccaro-Alamin, S., Foust, R., Vaithianathan, R., & Putnam-Hornstein, E. (2017). Risk assessment and decision making in child protective services: Predictive risk modeling in context. Children and Youth Services Review, 79, 291-298.
Related Project(s):
Allegheny Family Screening Tool, Douglas County Decision Aide, California Child Welfare Proof of Concept
Decomposing ethnic differences in university academic achievement in New Zealand
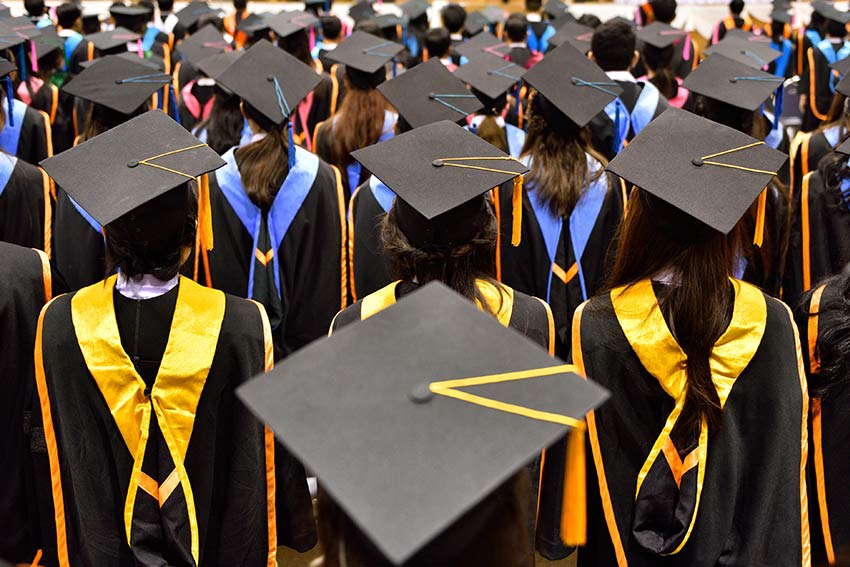
June-2017
Tim Maloney (CSDA Director 2016-2019) and Zhaoyi Cao use individual-level administrative data to examine the extent and potential explanations for the relatively poorer academic performance of three ethnic minority groups in their first year of study at a New Zealand university. Substantial differences in course completion rates and letter grades are found for Māori, Pasifika, and Asian students relative to their European counterparts. These large and significant gaps persist in the face of alternative definitions of ethnicity and sample restrictions.
Citation:
Cao, Z. & Maloney, T. (2017). Decomposing ethnic differences in university academic achievement in New Zealand. Higher Education, 75(4), 565-587.
Family Start Impact Study: Selected Extensions
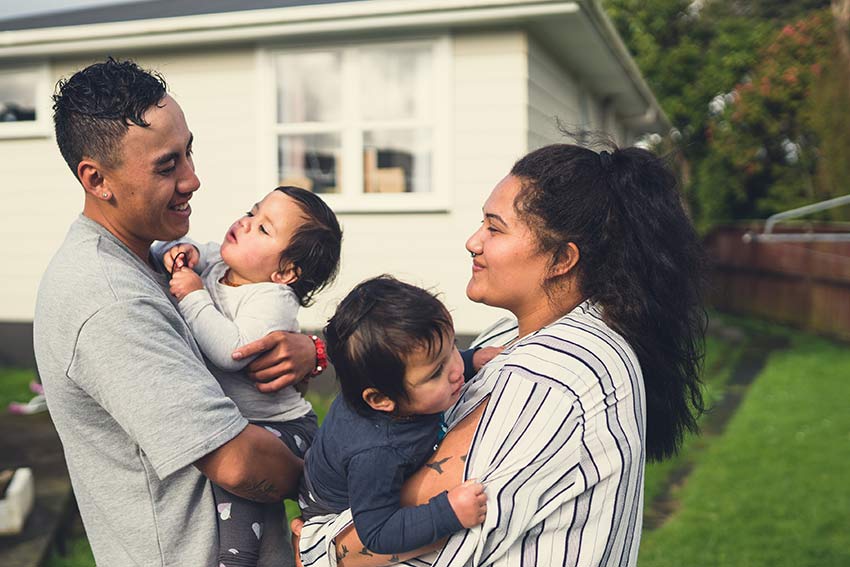
April-2017
This report by authors including Rhema Vaithianathan and Tim Maloney (CSDA Co-Director 2016-2019) provides selected extensions to a 2016 quasi-experimental evaluation of the Family Start Home Visiting programme. The extensions explore the efficacy of Family Start for additional sub-populations of participant families, and when delivered by sub-populations of providers. Results indicate that Family Start was effective in reducing some measures of post-neonatal infant mortality across sub-groups studied, including teen and non-teen mothers, children in families with and without past contact with Child Youth and Family, and Maori children receiving Family Start from Maori and mainstream providers.
Citation:
Vaithianathan, R., Wilson, M., Maloney, T., & Baird, S. (2017) Family Start Impact Study Selected Extensions Wellington: Ministry of Social Development.
Related Project:
Family Start Impact Evaluation
Developing predictive risk models to support child maltreatment hotline screening decisions: Allegheny County methodology and implementation
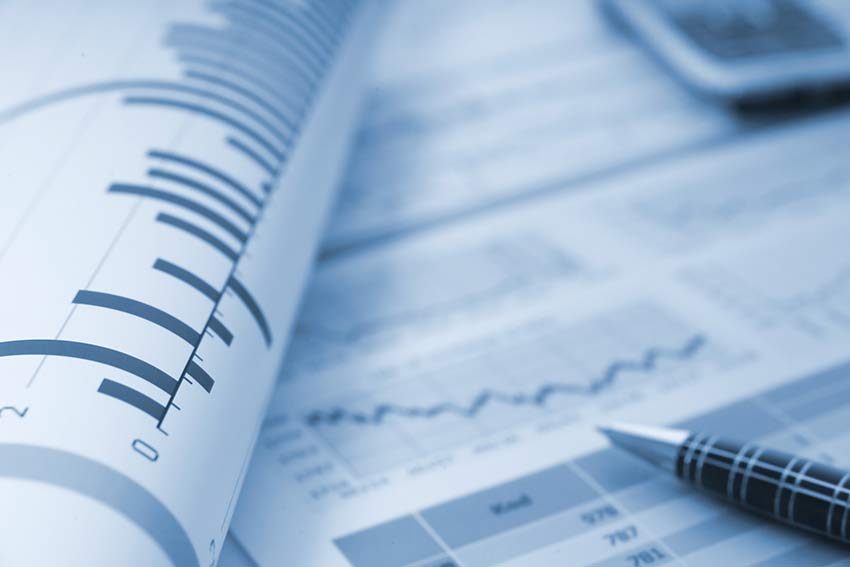
April-2017
This methodology document traces the development and implementation of the Allegheny Family Screening Tool (AFST), a child welfare predictive risk-modelling tool built by a research team led by Rhema Vaithianathan. The AFST is intended to support call screening of child maltreatment referrals by Allegheny County Department of Human Services. The methodology document includes an overview of existing practice, methodology and performance metrics of the model and details of the external validation and next steps for the model rebuild.
Citation:
Vaithianathan, R., Jiang, N., Maloney, T., Nand, P., & Putnam-Hornstein, E. (2017). Developing predictive risk models to support child maltreatment hotline screening decisions: Allegheny County methodology and implementation. Auckland: Centre for Social Data Analytics.
Related Project:
Allegheny Family Screening Tool, Douglas County Decision Aide
Black-White differences in child maltreatment reports and foster care placements: A statistical decomposition using linked administrative data
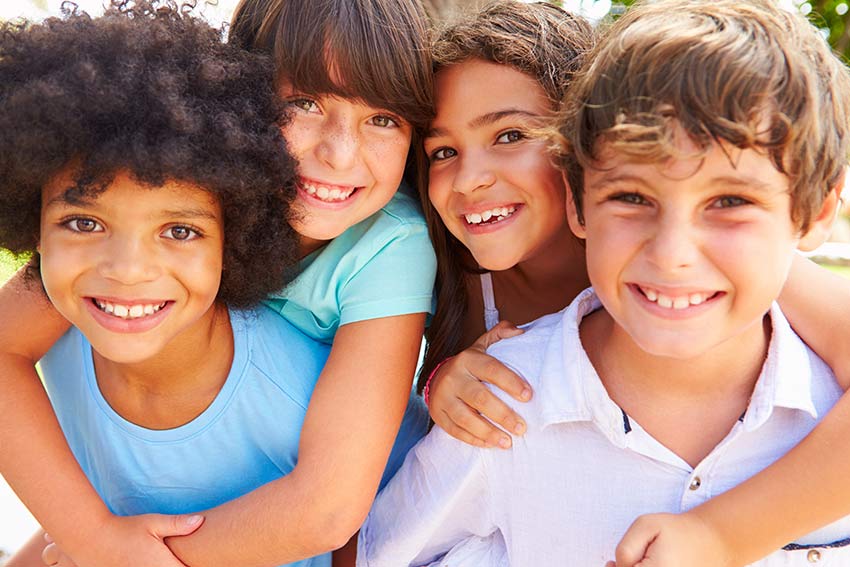
March-2017
The age and marital status of parents explains racial disparities in Child Protective Service (CPS) involvement, according to a study co-authored by Tim Maloney (CSDA Co-Director 2016-2019) and Rhema Vaithianathan. In the study, birth records for all children born in Allegheny County, Pennsylvania, between 2008 and 2010 were linked to administrative service records.
Citation:
Maloney, T., Jiang, N., Putnam-Hornstein, E., Dalton, E., & Vaithianathan, R. (2017). Black–White differences in child maltreatment reports and foster care placements: A statistical decomposition using linked administrative data. Maternal and child health journal, 21(3), 414-420.
Related Project:
Allegheny Family Screening Tool
Impact of School-based Support on Educational Outcomes of Teen-mothers: Evidence from New Zealand's "Teen Parent Units"
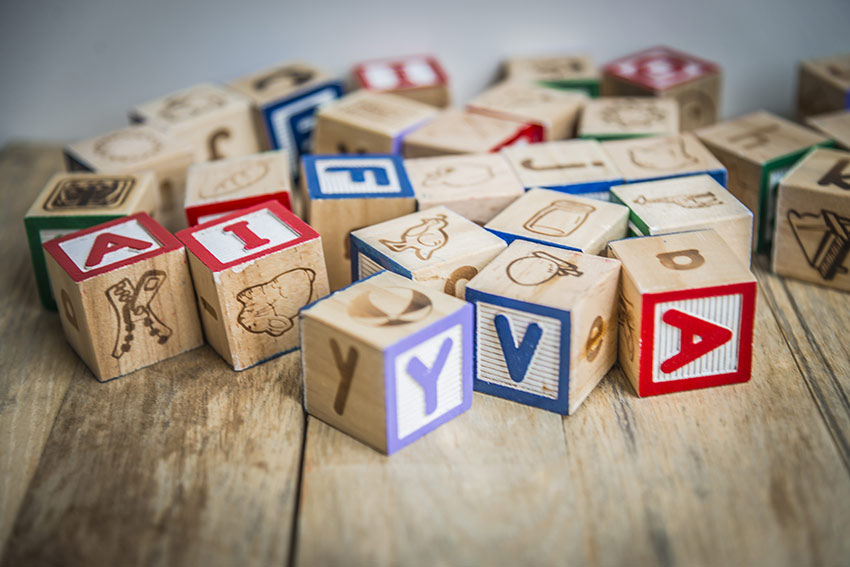
February-2017
Researchers including Rhema Vaithianathan and Tim Maloney (CSDA Co-Director 2016-2019) and found New Zealand teenage mothers with access to Teen Parent Units (TPUs) had better educational outcomes than those with no access. This research was conducted through the linkage of social sector administrative data.
Citation:
Vaithianathan, R., Maloney, T., Wilson, M., Staneva, A., & Jiang, N. (2017). Impact of School-based Support on Educational Outcomes of Teen-mothers: Evidence from New Zealand's "Teen Parent Units". Wellington: Ministry of Social Development.
Related Project:
Teen Parent Units Impact Evaluation
Impact of the Family Start Home Visiting Programme on Outcomes for Mothers and Children: A Quasi-Experimental Study
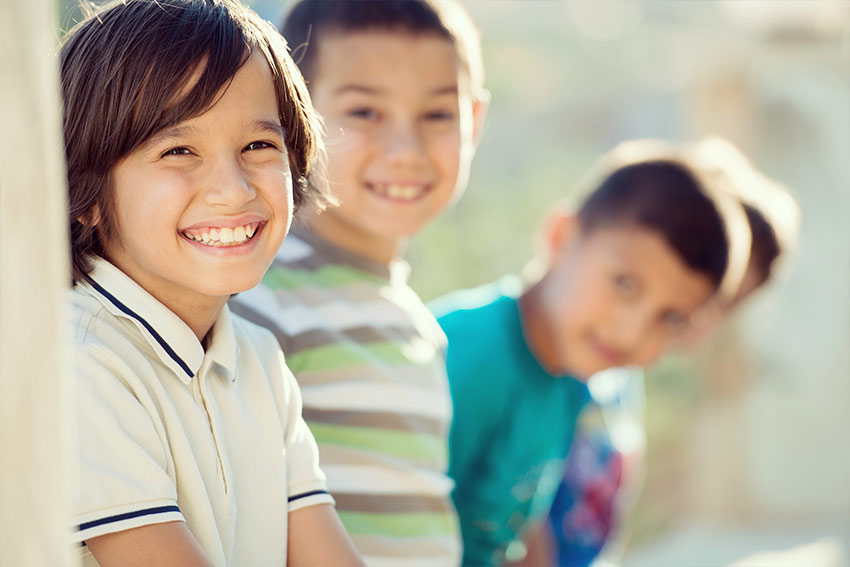
February-2016
Family Start workers make regular home visits and, using a structured program, seek to improve parenting capability and practice. Researchers including Rhema Vaithianathan and Tim Maloney (CSDA co-director 2016-2019)) evaluate the impact of the Family Start programme in improving outcomes for participating families.
Citation:
Vaithianathan, R., Wilson, M., Maloney, T., & Baird, S. (2016). The Impact of the Family Start Home Visiting Programme on Outcomes for Mothers and Children: A Quasi-Experimental Study. Wellington: Ministry of Social Development.
Related Project:
Family Start Impact Evaluation
Using predictive modelling to identify students at risk of poor university outcomes
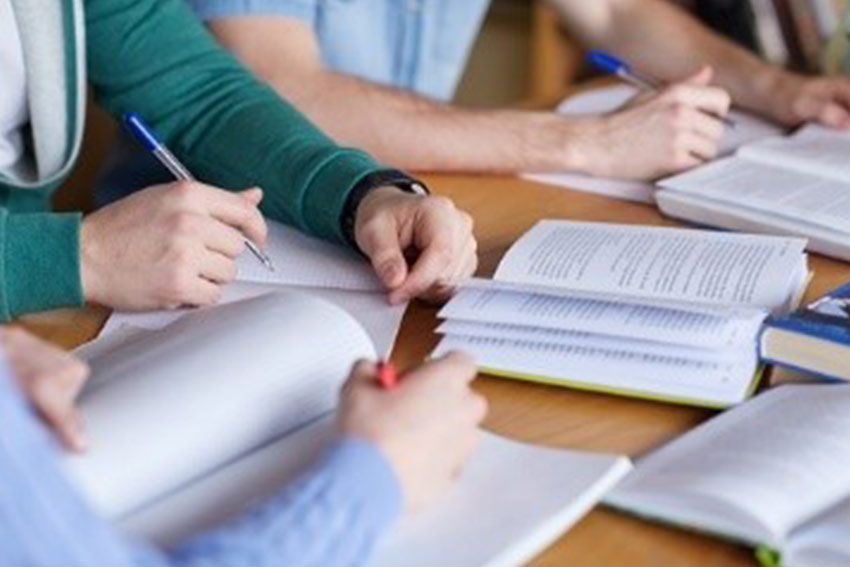
November-2014
This paper co-authored by Tim Maloney (CSDA co-director 2016-2019) outlines the use of predictive risk modelling tools to identify vulnerable students and understand factors that place these students at risk of non-completion and non-retention early in their university careers. Administrative data from the enrolment process is used to identify the factors contributing to these adverse outcomes.
Citation:
Jia, P., & Maloney, T. (2015). Using predictive modelling to identify students at risk of poor university outcomes. Higher Education, 70(1), 127-149.
Addressing child maltreatment in New Zealand: Is poverty reduction enough?
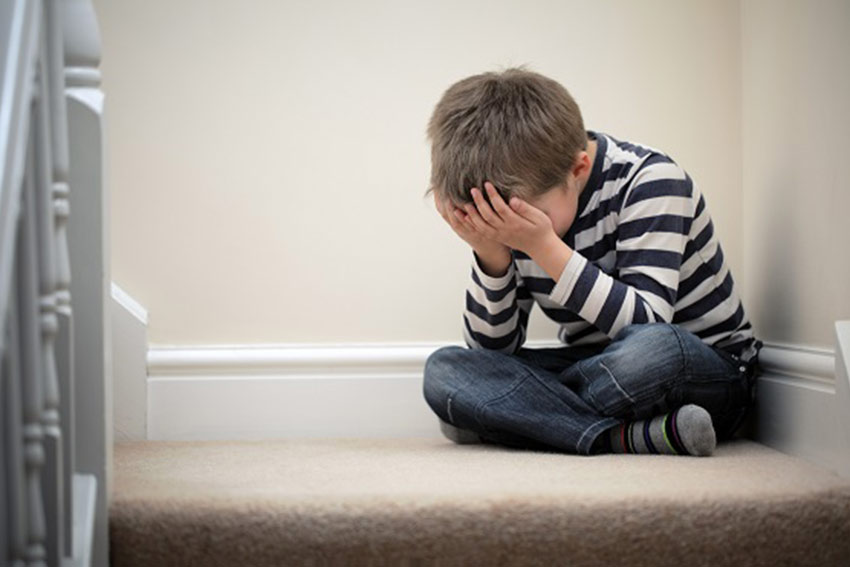
August-2014
This publication co-authored by Rhema Vaithianathan discusses the use of predictive risk models to target early and appropriate intervention to reduce child maltreatment and suggests potential issues that may arise from this approach to child protection.
Citation:
Dare, T., Vaithianathan, R., & De Haan, I. (2014). Addressing child maltreatment in New Zealand: is poverty reduction enough?. Educational Philosophy and Theory, 46(9), 989-994.
Integrating care for high risk patients in England using the virtual ward model
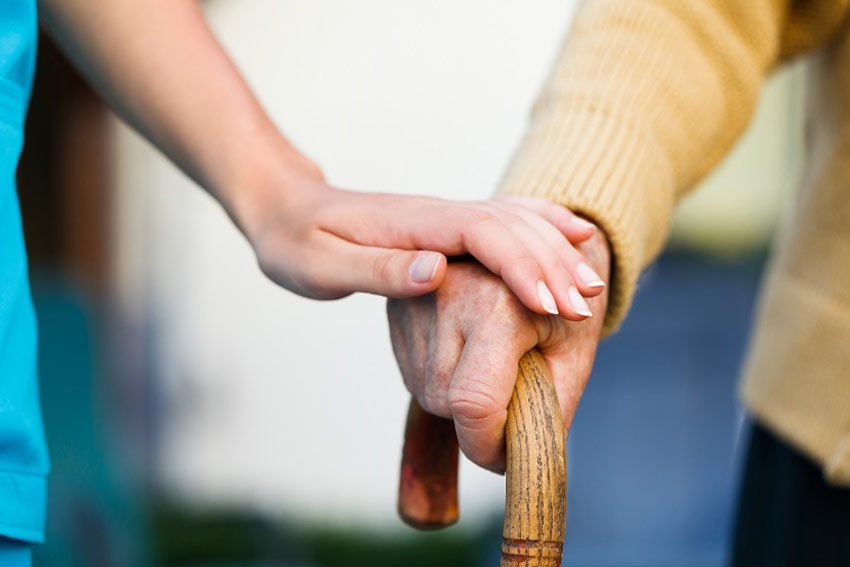
November-2013
This paper co-authored by Rhema Vaithianathan provides a discussion of the extent to which integrated care was achieved in the analysis of virtual wards in Croydon, Devon and Wandsworth. Key success factors and challenges are identified from the results of the case studies.
Citation:
Lewis, G., Vaithianathan, R., Wright, L., Brice, M., Lovell, P., Rankin, S., & Bardsley, M. (2013). Integrating care for high-risk patients in England using the virtual ward model: lessons in the process of care integration from three case sites. International Journal of Integrated Care, 13(4).
Children in the public benefit system at risk of maltreatment
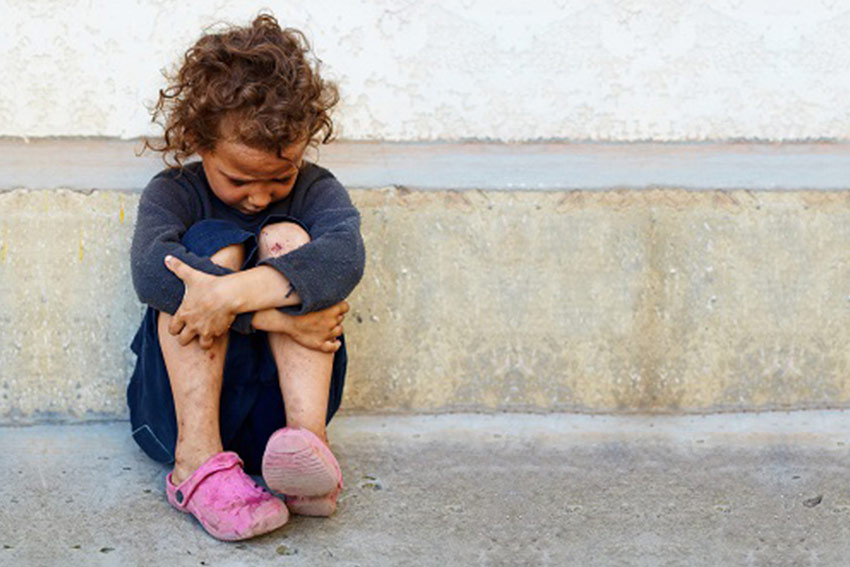
September-2013
This paper co-authored by Rhema Vaithianathan and Tim Maloney (CSDA co-director 2016-2019) outlines the method of using an automated predictive risk model to identify children at high risk of maltreatment, as well as discussing the strategic targeting of prevention activities toward these individuals.
Citation:
Vaithianathan, R., Maloney, T., Putnam-Hornstein, E., & Jiang, N. (2013). Children in the public benefit system at risk of maltreatment: Identification via predictive modeling. American Journal of Preventive Medicine, 45(3), 354-359.
Related Project(s):
Allegheny Family Screening Tool, Douglas County Decision Aide, California Child Welfare Predictive Risk Model Proof of Concept, Hello Baby Program