Measuring the working experience of doctors in training
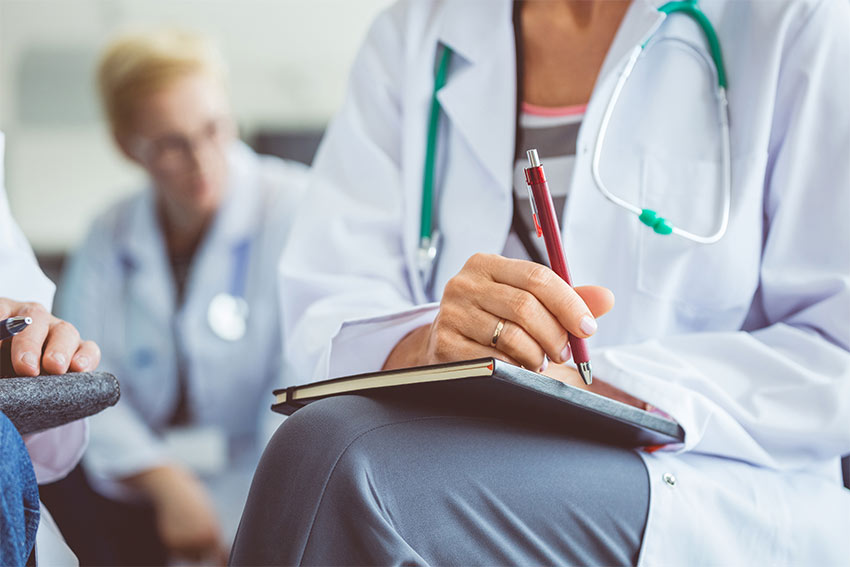
October-2020
In this paper published in the Future Healthcare Journal, researchers including CSDA’s Rhema Vaithianathan and Larissa Lorimer use an online tool (‘MyDay’) to look for an association between tasks and emotional affect for 565 doctors in training. They find that the participant trainee doctors spent a quarter of their time at work on paperwork or clinical work that did not involve patients, which was associated with more negative emotions. Positive emotions were associated with breaks, staff meetings, research, learning and clinical tasks that involved patients. Trainee doctors reporting that they had considered leaving medicine reported more negative feelings. The authors conclude that systematic workplace changes, like regular breaks, reduced paperwork and improved IT systems could contribute to positive workplace experiences and reduce the intention to quit among doctors in training.
Citation:
Hockey, P., Vaithianathan, R., Baeker, A., Beer, F., Goodall, A. H., Hammerton, M., Jarvis, R., Brock, S., & Lorimer, L. (2020). Measuring the working experience of doctors in training. Future Healthcare Journal, 7(3), e17–e22. https://doi.org/10.7861/fhj.2020-0005
Related projects:
MyDay Survey with Health Education England, Working Across Wessex
Implementing the Hello Baby Prevention Program in Allegheny County Methodology Report
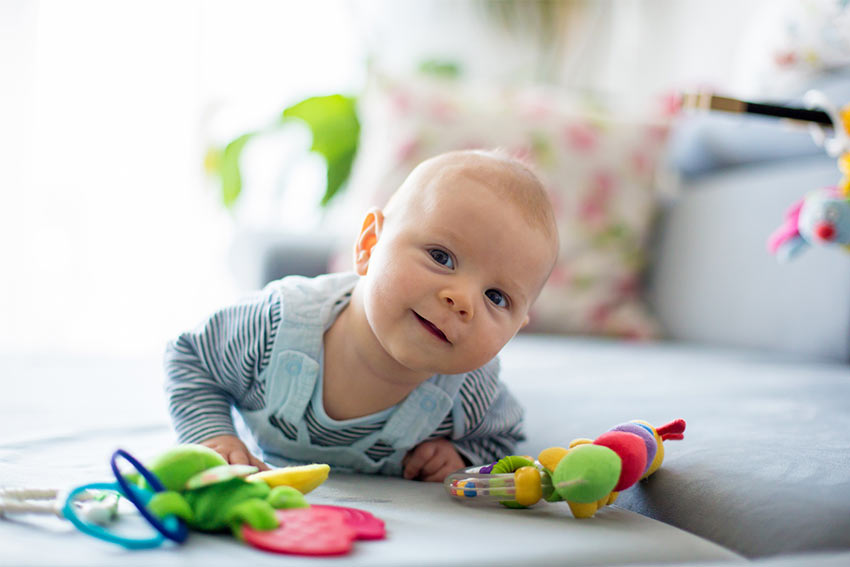
September-2020
This report summarises the development and implementation of the Hello Baby program which is supported by a predictive risk modelling (PRM) tool, built and deployed by the Centre for Social Data Analytics. Hello Baby is a new universal tiered program designed to prevent harm and protect children, introduced as a pilot program by the Allegheny County Department of Human Services in September 2020. The County will use the PRM tool to establish eligibility for higher intensity services, alongside self-referral and professional referral. The methodology provides background to the introduction of Hello Baby, explains the selection of the PRM tool as an eligibility pathway, describes how historical data was used to train and validate the PRM tool and explains how the tool will be used as part of the implemented Hello Baby program. Additional documentation about the Hello Baby program including ethics reports and FAQs is found on the Allegheny County website.
Citation:
Vaithianathan, Rhema; Diana Benavides-Prado and Emily Putnam-Hornstein. Implementing the Hello Baby Prevention Program in Allegheny County. Centre for Social Data Analytics. Auckland, New Zealand. September 2020.
Related projects:
Hello Baby Program - Proactive Child Welfare Predictive Risk Model
Using Predictive Risk Modeling to Prioritize Services for People Experiencing Homelessness in Allegheny County: Methodology Paper for the Allegheny Housing Assessment
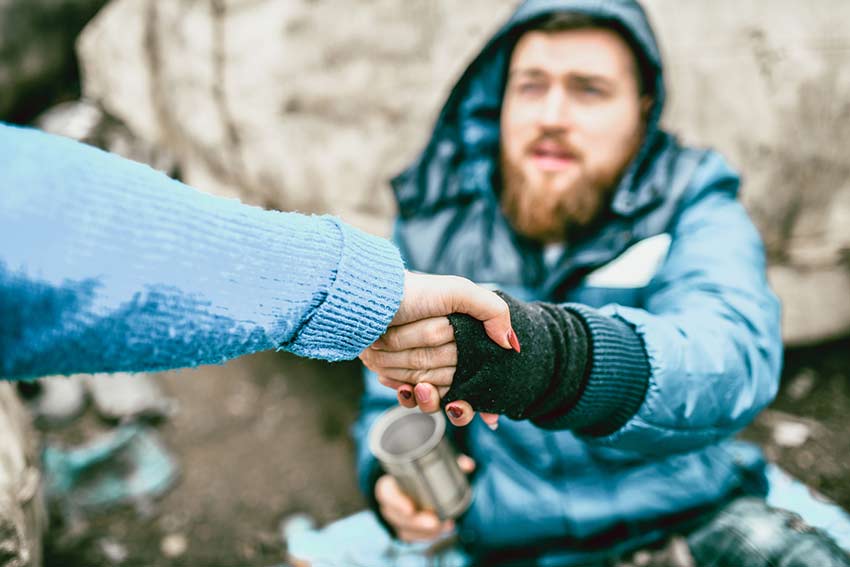
September-2020
This report summarises the development and implementation of the Allegheny Housing Assessment, a predictive risk modelling tool, built and deployed by the Centre for Social Data Analytics for the Allegheny County Department of Human Services, Pennsylvania, United States. The AHA will be used to support the prioritisation of people for longer term housing programs. A prioritisation tool is needed because the demand for housing exceeds supply. The AHA replaces the VI-SPDAT, a prioritisation system without local validation. The methodology provides background to the development of the AHA including a comparison between the AHA and the previously used VI-SPDAT. The methodology provides background on homeless services in Allegheny County and the decision to explore the use of a predictive risk modelling tool, before discussing elements including community engagement, the methodology used to develop the tool, accuracy and the business process it will be used within.
Citation:
Vaithianathan, R. & Kithulgoda, C.I. (2020). Using Predictive Risk Modeling to Prioritize Services for People Experiencing Homelessness in Allegheny County. Centre for Social Data Analytics. Auckland, New Zealand.
Related projects:
Hospital Injury Encounters of Children Identified by a Predictive Risk Model for Screening Child Maltreatment Referrals: Evidence from the Allegheny Family Screening Tool
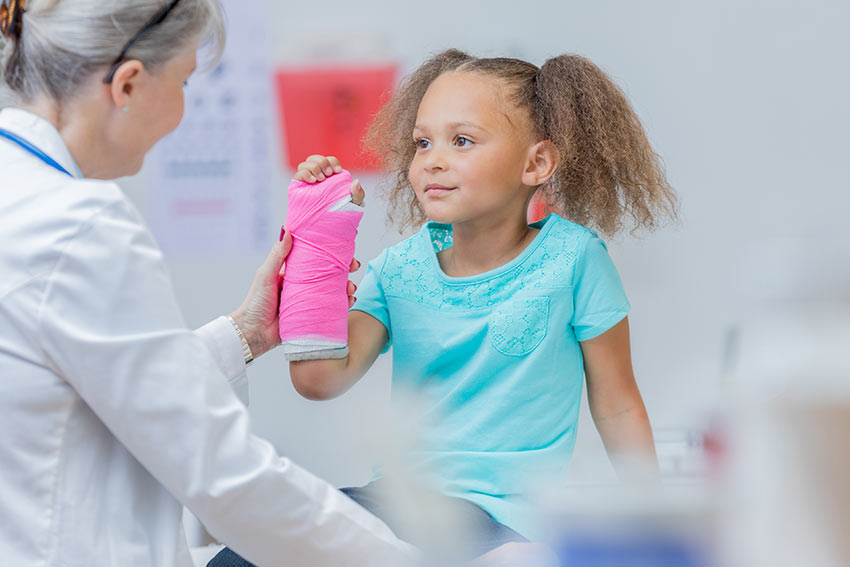
August-2020
This paper confirms that children identified as at risk by the Allegheny Family Screening Tool (AFST), a predictive risk modelling tool that supports child protection decisions in Allegheny County (PA, United States), are also at considerably heightened risk of hospitalisation injury. The authors, including Rhema Vaithianathan and Diana Benavides Prado, used a one-off linkage between the child protection system and Pittsburgh Children’s Hospital data to show that children identified as at risk by the AFST are indeed at considerably heightened risk of injury, abuse, and self-harm hospitalisation. The authors also identified a sharply heightened hospitalisation risk for children receiving the highest AFST scores and found that the AFST is particularly sensitive to the risk facing White children, which means the child protection system is potentially under protecting those children.
Citation:
Vaithianathan R, Putnam-Hornstein E, Chouldechova A, Benavides-Prado D, Berger R. Hospital Injury Encounters of Children Identified by a Predictive Risk Model for Screening Child Maltreatment Referrals: Evidence From the Allegheny Family Screening Tool. JAMA Pediatr. Published online August 03, 2020. doi:10.1001/jamapediatrics.2020.2770
Related projects:
Allegheny Family Screening Tool
Digital Contact Tracing for COVID-19: A Primer for Policymakers
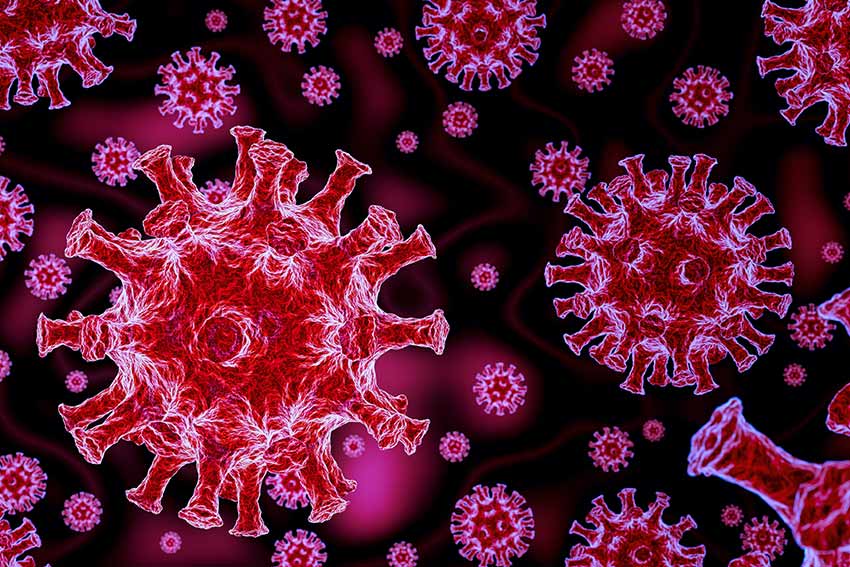
June-2020
This working paper is intended as a guide for policymakers considering the use of digital contact tracing solutions for Covid-19. The authors, including Rhema Vaithianathan and Nina Anchugina, use a simple graphical model of infection transmissions to illustrate why COVID-19 is particularly challenging to manage with traditional manual contact tracing. They find that digital contact tracing solutions for COVID-19 must offer exceptional speed and achieve high take-up rates to be useful. The paper discusses the importance of social licence for take-up, and explains how a commitment to impact evaluation can help build trust.
Citation:
Vaithianathan, R., Ryan, M., Anchugina, N., Selvey, L., Dare, T., & Brown, A. (2020). Digital Contact Tracing for Covid-19: A Primer for Policymakers (Working Paper). Centre for Social Data Analytics: Auckland University of Technology & The University of Queensland.
Related links
- 'Covid-19 Contact Tracing Apps: What works?'
presentation by Nina Anchugina for the Chief Data and Analytics Officer (CDAO) NZ conference, November 2020 (video) - COVIDSafe Still Has Crucial iPhone Issues Nearly 2 Months Later
Gizmodo, 16 June 2020. - Even 100% COVIDSafe Uptake Won’t Make It Effective, Researcher Says
Gizmodo, 11 June 2020. - COVIDSafe extremely limited’: New research
Innovation Aus, 9 June 2020. - Five ways New Zealand can keep Covid-19 cases at zero
The Guardian, 8 June 2020. - Trust in COVID Tracer app needs to be built to see sufficient uptake - Anna Brown
Newshub, 7 June 2020 - Covid 19 coronavirus: The three extra steps NZ needs to take at alert level 1
NZ Herald, 2 June 2020. - Coronavirus: Government app lacking crucial features – academic
Radio NZ, 21 May 2020. - Covid 19 coronavirus: Govt tracing app only good for 'one unlikely scenario' – academic
NZ Herald, 20 May 2020. - Covid 19 coronavirus: Rhema Vaithianathan: NZ is the Wild West of contact tracing
NZ Herald, 14 May 2020.
Towards Knowledgeable Supervised Lifelong Learning Systems
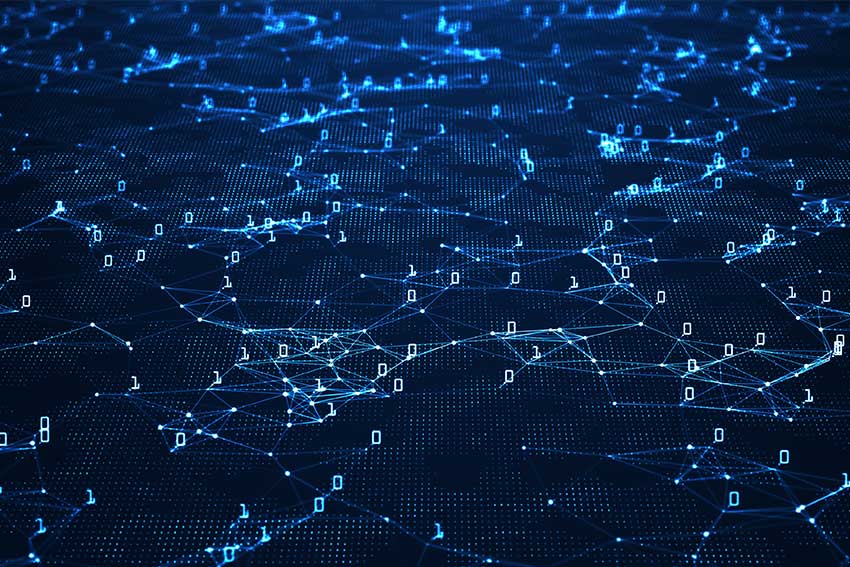
May-2020
This paper co-authored by Diana Benavides-Prado offers a potential solution to challenges associated with machine learning systems that learn sequentially. The authors propose a framework for long-term learning systems (Proficiente), which relies on transferring knowledge across tasks in two directions using Support Vector Machines (SVM). Proficiente makes it possible to transfer knowledge acquired from a previous task forward to a new target task and to transfer knowledge acquired from recent tasks backward to refine knowledge acquired from previous tasks. The authors demonstrate that transferring selected knowledge forward and backwards has the potential to encourage learning systems to become more knowledgeable while observing tasks sequentially.
Citation:
Benavides-Prado, D., Koh, Y. S., & Riddle, P. (2020). Towards Knowledgeable Supervised Lifelong Learning Systems. Journal of Artificial Intelligence Research, 68, 159-224.