Research offers new framework to help machine learning systems learn over time
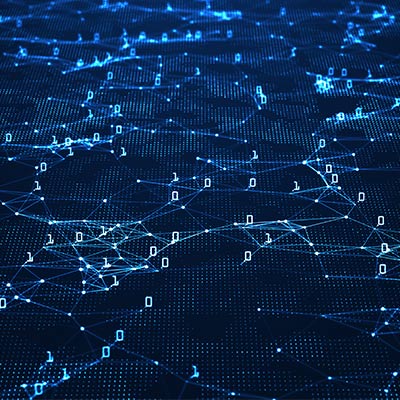
New research co-authored by Diana Benavides Prado, a senior research fellow at CSDA, proposes a framework designed to improve the knowledge acquired by machine learning systems that learn sequentially (by observing learning tasks one at a time).
The framework, which is called Proficiente, transfers knowledge selectively between tasks using Support Vector Machines (SVM).
To date, research about lifelong and continual machine learning has mainly focused on learning new tasks better and avoiding catastrophic forgetting of knowledge of previous tasks. This paper explores the possibility of also refining existing knowledge, which may help improve the performance of an entire learning system.
Benavides-Prado co-authored “Towards Knowledgeable Supervised Lifelong Learning Systems”, published in the Journal of Artificial Intelligence Research, with Yun Sing Koh and Patricia Riddle from The University of Auckland.
The authors demonstrate that transferring selected knowledge forward to new tasks and backward to existing models (representing previous tasks) has the potential to encourage learning systems to become more knowledgeable (more accurate) while observing tasks sequentially.
“A machine learning system should become more knowledgeable as more related tasks are learned, just like a human” says Benavides-Prado. “When tasks are observed one at a time (rather than all at the same point in time) opportunities to transfer knowledge need to be intentionally built into the process. This will not only encourage systems to learn new tasks faster and better, like in classic transfer learning, but also means that old tasks learned with data available at the time can potentially be refined as new data is observed in related incoming tasks.”
“Lifelong and continual machine learning have become remarkably important as an attempt to imitate the human continuous process of learning tasks sequentially. Lifelong learning systems like those used by self-driving cars, where the environment is highly changeable, and conversation systems that need to learn new words and semantics while interacting with humans, can benefit greatly from advances in these areas.”
Benavides-Prado will present a summary of the paper at the 2020 International Conference on Machine Learning Workshop in Continual Learning (July 2020).